Thanks to the almost 6000 folks joining us! We are still working on launching the podcast, and the Latent Space Hackathon has been 7x oversubscribed. We’ll be taking some sponsors and expanding our Demo Day, so join us if you will have something to share in SF on Feb 17! See you there!
Why do blatantly untrue lies about AI spread further and faster than truths?
You’ve heard the rumors about GPT-4 having more parameters than there are stars in the sky, grains of sand on the beach, and drops of water in the ocean. You’ve seen the Small Circle, Big Circle memes from the hustle bros too smoothbrained or intellectually dishonest to understand Chinchilla results.
>4 million people saw the lie (probably way more), but less than 68k people heard Sam Altman debunk it as “complete bullshit” (thanks to reader Nik for correcting link).
But it isn’t limited to hustle bros - even Wharton professors are getting into it, claiming that ChatGPT “clearly passes” medical, legal and MBA exams, only clarifying his overstatement “in thread”.
again, >4m saw the overstatement, diligent corrections get nothing.
The latest in irresponsible #foomerism this week is lies about ChatGPT user numbers, which two publications estimated at 100m users (UBS/Reuters) and 5m users (Forbes) on the same day.
Forbes’ reporting involved two reporters spending multiple days embedding themselves in the OpenAI offices and interviewing more than 60 people, including two OpenAI founders.
UBS’ estimate involved some guy opening Similarweb for 2 minutes (an inexact estimate at best) and wildly extrapolating daily traffic numbers.
Guess which number went viral, presented as fact?
It’s not new that people only read headlines, or that a lie will run around the world before the truth can get its pants on. But there is something about AI that makes it inherently susceptible to suspension of disbelief. Perhaps this is built into the architecture of deep learning - involving many hidden layers and bitter lessons that defy human intelligent design.
The Origins of #Foomerism
I’ve taken to calling this behavior #foomerism, an ironic malapropism that reflects a third extreme playing on doomerism (AGI fatalists) and FOMO (hustle bros). The hashtag #foom itself has been gaining steam from techno-optimists reflecting the onomatopoetry of the “slowly, then all at once” nature of exponential growth:


The nature of AI discourse tends to be as binary as the activation functions on which it is based - either you are a #foomer techno-optimist, or you are a AGI-fearing Luddite, with no position in between. The #foomer in chief of the Noughties was probably Ray Kurzweil, who coined the awkward name of The Singularity, and the Tens brought us Tim Urban, who was much better at illustrating:

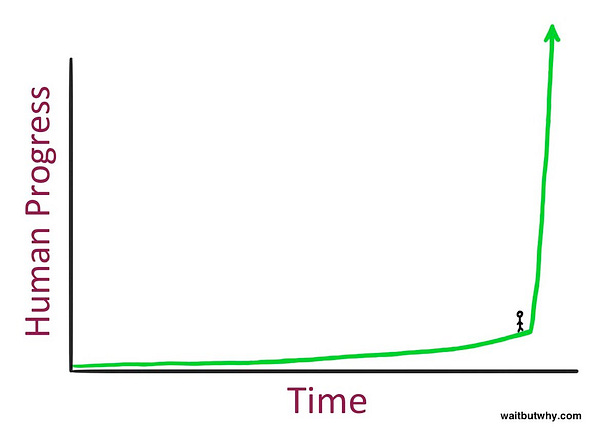
The Long AI Summer of the roaring 2020’s have finally democratized #foomerism, and now finally everybody is free to repost big circle small circle JPEGs spreading ideas from big brains to smaller.
AI Moloch and #Foomerism
We saw the beginnings of AI Moloch in January, when Sundar Pichai hit the Code Red. Now they have laid off 12,000 people (including 36 Massage Therapist Level II’s) and Larry and Sergey are back committing code.
AI Moloch is now spreading to social media as shameless #foomerism. In response to my observation about the 20x differences in ChatGPT user estimate, Forbes’ Alex Konrad admitted their estimate was “likely conservative”, but how likely do you think he will want to maintain his conservatism given his incentives and the clear results?
Don’t hate the players, hate the game, right?
And so the hustle bro fistpumps at getting a “hit”.
The Ivy League professor wilfully overstates paper results.
The career reporter kicks himself for being outdone by a faceless bank analyst.
Fundamentally, we suspend our disbelief on AI, and knowingly retweet lies and half-truths about AI, because deep down we want to be lied to. We want Santa to deliver gifts, we want the tooth fairy swapping our teeth for coin, we want deontological absolutes with no utilitarian consequences. We want magic in our lives, we want to see superhuman feats, we want to have been there for the major advances in human civilization.
I assume you’re still reading this boring essay, bereft of wild overstatements and unfounded speculations on the future, because you are interested in staying somewhat close to practical reality.
How can I “keep it real”, you ask?
Exponential #foomers don’t talk about S-curves
We have in fact lived through another #foom in recent years. Another time when the “you just don’t understand exponentials” people talked down to the unwashed uneducated masses too stupid to look up from their phones.
Remember Covid?
Every day we retweeted and obsessed over big numbers going up. These same people stopped commenting when numbers stopped going up as much, and were completely silent when they started going down. Piping back up on each aftershock, but in fewer numbers and less stringently each time.
I write this not to question aggressive pre-emptive Covid action, which of course had the better risk-reward, but to point out that the more nuanced and harder-to-meme reality is acknowledging that exponential curves do exist, but they also tap out, and run into invisible asymptotes and real-world limits, and they are also extremely hard to forecast. By far my favorite visualization of this is from systems modeler Dr. Constance Crozier (play the video and imagine yourself getting excited The Singularity on the way up and then falling silent as the asymptote arrives):

S-curves are everywhere in tech. Both Fred Wilson and Marc Andreesen are paricularly fond of the Carlota Perez Framework, which I have written about in my prior blogposts and book. Every technology has a #foom period and then a more “boring” (but profitable) maturity phase, the layers of civilized technology growth alternating neatly between invention and infrastructure.
Multiple s-curves can daisy chain in an unending but undulating tapestry of human progress (thanks to CRV’s Brittany Walker for pointing me to this):
Next time you run into a #foomer, ask them about S-curves.
If they don’t know, show this to them and ask about limits and practical constraints.
And if they don’t care: run.
I'd say presumption that it'll level out before society is completely disrupted is more shameless.
https://gwern.net/scaling-hypothesis
> GPT-3 in 2020 makes as good a point as any to take a look back on the past decade. It’s remarkable to reflect that someone who started a PhD because they were excited by these new “ResNets” would still not have finished it by now—that is how recent even resnets are, never mind Transformers, and how rapid the pace of progress is. In 2010, one could easily fit everyone in the world who genuinely believed in deep learning into a moderate-sized conference room (assisted slightly by the fact that 3 of them were busy founding DeepMind).
> In 2010, who would have predicted that over the next 10 years, deep learning would undergo a Cambrian explosion causing a mass extinction of alternative approaches throughout machine learning, that models would scale up to 175,000 million parameters, and that these enormous models would just spontaneously develop all these capabilities?
> No one. That is, no one aside from a few diehard connectionists written off as willfully-deluded old-school fanatics by the rest of the AI community (never mind the world), such as Moravec, Schmidhuber, Sutskever, Legg, & Amodei? One of the more shocking things about looking back is realizing how unsurprising and easily predicted all of this was if you listened to the right people. In 1998, 22 years ago, Moravec noted that AI research could be deceptive... “AI research must wait for the power to become more affordable.”
> Affordable meaning a workstation roughly ~$1,930 ($1,000 in 1998); sufficiently cheap compute to rival a human would arrive sometime in the 2020s, with the 2010s seeing affordable systems in the lizard–mouse range.
> As it happens, the start of the DL revolution is typically dated to AlexNet in 2012, by a grad student using 2 GTX 580 3GB GPUs (launch list price of $683 ($500 in 2010), for a system build cost of perhaps $1,979 ($1,500 in 2012). 2020 saw GPT-3 arrive, and as discussed before, there are many reasons to expect the cost to fall, in addition to the large hardware compute gains that are being forecast for the 2020s despite the general deceleration of Moore’s law
> The accelerating pace of the last 10 years should wake anyone from their dogmatic slumber and make them sit upright. And there are 28 years left in Moravec’s forecast…
> Even in 2015, the scaling hypothesis seemed highly dubious: you needed something to scale, after all, and it was all too easy to look at flaws in existing systems and imagine that they would never go away and progress would sigmoid any month now, soon.
> ...the future arrived at first slowly and then quickly. Yet, here we are: all honor to the fanatics, shame and humiliation to the critics! If only one could go back 10 years, or even 5, to watch every AI researchers’ head explode reading this paper… Unfortunately, few heads appear to be exploding now, because human capacity for hindsight & excuses is boundless
I’m gonna steal “foomerism” on my own Substack. This is spot on.