Our next 2 big events are AI UX and the World’s Fair. Join and apply to speak/sponsor!
Due to timing issues we didn’t have an interview episode to share with you this week, but not to worry, we have more than enough “weekend special” content in the backlog for you to get your Latent Space fix, whether you like thinking about the big picture, or learning more about the pod behind the scenes, or talking Groq and GPUs, or AI Leadership, or Personal AI.
Enjoy!
AI Breakdown
The indefatigable NLW had us back on his show for an update on the Four Wars, covering Sora, Suno, and the reshaped GPT-4 Class Landscape:
and a longer segment on AI Engineering trends covering the future LLM landscape (Llama 3, GPT-5, Gemini 2, Claude 4), Open Source Models (Mistral, Grok), Apple and Meta’s AI strategy, new chips (Groq, MatX) and the general movement from baby AGIs to vertical Agents:
Thursday Nights in AI
We’re also including swyx’s interview with Josh Albrecht and Ali Rohde to reintroduce swyx and Latent Space to a general audience, and engage in some spicy Q&A:
Dylan Patel on Groq
We hosted a private event with Dylan Patel of SemiAnalysis (our last pod here):
Not all of it could be released so we just talked about our Groq estimates:
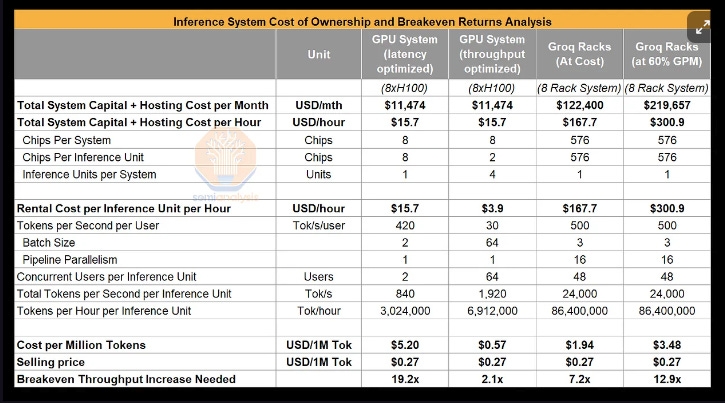
Milind Naphade - Capital One
In relation to conversations at NeurIPS and Nvidia GTC and upcoming at World’s Fair, we also enjoyed chatting with Milind Naphade about his AI Leadership work at IBM, Cisco, Nvidia, and now leading the AI Foundations org at Capital One. We covered:
Milind’s learnings from ~25 years in machine learning
Lessons from working with Jensen Huang for 6 years and being CTO of Metropolis
Thoughts on relevant AI research
GTC takeaways and what makes NVIDIA special
If you’d like to work on building solutions rather than platform (as Milind put it), his Applied AI Research team at Capital One is hiring, which falls under the Capital One Tech team.
Personal AI Meetup
It all started with a meme:
Within days of each other, BEE, FRIEND, EmilyAI, Compass, Nox and LangFriend were all launching personal AI wearables and assistants. So we decided to put together a the world’s first Personal AI meetup featuring creators and enthusiasts of wearables. The full video is live now, with full show notes within.
Timestamps
[00:01:13] AI Breakdown Part 1
[00:02:20] Four Wars
[00:13:45] Sora
[00:15:12] Suno
[00:16:34] The GPT-4 Class Landscape
[00:17:03] Data War: Reddit x Google
[00:21:53] Gemini 1.5 vs Claude 3
[00:26:58] AI Breakdown Part 2
[00:27:33] Next Frontiers: Llama 3, GPT-5, Gemini 2, Claude 4
[00:31:11] Open Source Models - Mistral, Grok
[00:34:13] Apple MM1
[00:37:33] Meta's $800b AI rebrand
[00:39:20] AI Engineer landscape - from baby AGIs to vertical Agents
[00:47:28] Adept episode - Screen Multimodality
[00:48:54] Top Model Research from January Recap
[00:53:08] AI Wearables
[00:57:26] Groq vs Nvidia month - GPU Chip War
[01:00:31] Disagreements
[01:02:08] Summer 2024 Predictions
[01:04:18] Thursday Nights in AI - swyx
[01:33:34] Dylan Patel - Semianalysis + Latent Space Live Show
[01:34:58] Groq
Transcript
[00:00:00] swyx: Welcome to the Latent Space Podcast Weekend Edition. This is Charlie, your AI co host. Swyx and Alessio are off for the week, making more great content. We have exciting interviews coming up with Elicit, Chroma, Instructor, and our upcoming series on NSFW, Not Safe for Work AI. In today's episode, we're collating some of Swyx and Alessio's recent appearances, all in one place for you to find.
[00:00:32] swyx: In part one, we have our first crossover pod of the year. In our listener survey, several folks asked for more thoughts from our two hosts. In 2023, Swyx and Alessio did crossover interviews with other great podcasts like the AI Breakdown, Practical AI, Cognitive Revolution, Thursday Eye, and Chinatalk, all of which you can find in the Latentspace About page.
[00:00:56] swyx: NLW of the AI Breakdown asked us back to do a special on the 4Wars framework and the AI engineer scene. We love AI Breakdown as one of the best examples Daily podcasts to keep up on AI news, so we were especially excited to be back on Watch out and take
[00:01:12] NLW: care
[00:01:13] AI Breakdown Part 1
[00:01:13] NLW: today on the AI breakdown. Part one of my conversation with Alessio and Swix from Latent Space.
[00:01:19] NLW: All right, fellas, welcome back to the AI Breakdown. How are you doing? I'm good. Very good. With the last, the last time we did this show, we were like, oh yeah, let's do check ins like monthly about all the things that are going on and then. Of course, six months later, and, you know, the, the, the world has changed in a thousand ways.
[00:01:36] NLW: It's just, it's too busy to even, to even think about podcasting sometimes. But I, I'm super excited to, to be chatting with you again. I think there's, there's a lot to, to catch up on, just to tap in, I think in the, you know, in the beginning of 2024. And, and so, you know, we're gonna talk today about just kind of a, a, a broad sense of where things are in some of the key battles in the AI space.
[00:01:55] NLW: And then the, you know, one of the big things that I, that I'm really excited to have you guys on here for us to talk about where, sort of what patterns you're seeing and what people are actually trying to build, you know, where, where developers are spending their, their time and energy and, and, and any sort of, you know, trend trends there, but maybe let's start I guess by checking in on a framework that you guys actually introduced, which I've loved and I've cribbed a couple of times now, which is this sort of four wars of the, of the AI stack.
[00:02:20] Four Wars
[00:02:20] NLW: Because first, since I have you here, I'd love, I'd love to hear sort of like where that started gelling. And then and then maybe we can get into, I think a couple of them that are you know, particularly interesting, you know, in the, in light of
[00:02:30] swyx: some recent news. Yeah, so maybe I'll take this one. So the four wars is a framework that I came up around trying to recap all of 2023.
[00:02:38] swyx: I tried to write sort of monthly recap pieces. And I was trying to figure out like what makes one piece of news last longer than another or more significant than another. And I think it's basically always around battlegrounds. Wars are fought around limited resources. And I think probably the, you know, the most limited resource is talent, but the talent expresses itself in a number of areas.
[00:03:01] swyx: And so I kind of focus on those, those areas at first. So the four wars that we cover are the data wars, the GPU rich, poor war, the multi modal war, And the RAG and Ops War. And I think you actually did a dedicated episode to that, so thanks for covering that. Yeah, yeah.
[00:03:18] NLW: Not only did I do a dedicated episode, I actually used that.
[00:03:22] NLW: I can't remember if I told you guys. I did give you big shoutouts. But I used it as a framework for a presentation at Intel's big AI event that they hold each year, where they have all their folks who are working on AI internally. And it totally resonated. That's amazing. Yeah, so, so, what got me thinking about it again is specifically this inflection news that we recently had, this sort of, you know, basically, I can't imagine that anyone who's listening wouldn't have thought about it, but, you know, inflection is a one of the big contenders, right?
[00:03:53] NLW: I think probably most folks would have put them, you know, just a half step behind the anthropics and open AIs of the world in terms of labs, but it's a company that raised 1. 3 billion last year, less than a year ago. Reed Hoffman's a co founder Mustafa Suleyman, who's a co founder of DeepMind, you know, so it's like, this is not a a small startup, let's say, at least in terms of perception.
[00:04:13] NLW: And then we get the news that basically most of the team, it appears, is heading over to Microsoft and they're bringing in a new CEO. And you know, I'm interested in, in, in kind of your take on how much that reflects, like hold aside, I guess, you know, all the other things that it might be about, how much it reflects this sort of the, the stark.
[00:04:32] NLW: Brutal reality of competing in the frontier model space right now. And, you know, just the access to compute.
[00:04:38] Alessio: There are a lot of things to say. So first of all, there's always somebody who's more GPU rich than you. So inflection is GPU rich by startup standard. I think about 22, 000 H100s, but obviously that pales compared to the, to Microsoft.
[00:04:55] Alessio: The other thing is that this is probably good news, maybe for the startups. It's like being GPU rich, it's not enough. You know, like I think they were building something pretty interesting in, in pi of their own model of their own kind of experience. But at the end of the day, you're the interface that people consume as end users.
[00:05:13] Alessio: It's really similar to a lot of the others. So and we'll tell, talk about GPT four and cloud tree and all this stuff. GPU poor, doing something. That the GPU rich are not interested in, you know we just had our AI center of excellence at Decibel and one of the AI leads at one of the big companies was like, Oh, we just saved 10 million and we use these models to do a translation, you know, and that's it.
[00:05:39] Alessio: It's not, it's not a GI, it's just translation. So I think like the inflection part is maybe. A calling and a waking to a lot of startups then say, Hey, you know, trying to get as much capital as possible, try and get as many GPUs as possible. Good. But at the end of the day, it doesn't build a business, you know, and maybe what inflection I don't, I don't, again, I don't know the reasons behind the inflection choice, but if you say, I don't want to build my own company that has 1.
[00:06:05] Alessio: 3 billion and I want to go do it at Microsoft, it's probably not a resources problem. It's more of strategic decisions that you're making as a company. So yeah, that was kind of my. I take on it.
[00:06:15] swyx: Yeah, and I guess on my end, two things actually happened yesterday. It was a little bit quieter news, but Stability AI had some pretty major departures as well.
[00:06:25] swyx: And you may not be considering it, but Stability is actually also a GPU rich company in the sense that they were the first new startup in this AI wave to brag about how many GPUs that they have. And you should join them. And you know, Imadis is definitely a GPU trader in some sense from his hedge fund days.
[00:06:43] swyx: So Robin Rhombach and like the most of the Stable Diffusion 3 people left Stability yesterday as well. So yesterday was kind of like a big news day for the GPU rich companies, both Inflection and Stability having sort of wind taken out of their sails. I think, yes, it's a data point in the favor of Like, just because you have the GPUs doesn't mean you can, you automatically win.
[00:07:03] swyx: And I think, you know, kind of I'll echo what Alessio says there. But in general also, like, I wonder if this is like the start of a major consolidation wave, just in terms of, you know, I think that there was a lot of funding last year and, you know, the business models have not been, you know, All of these things worked out very well.
[00:07:19] swyx: Even inflection couldn't do it. And so I think maybe that's the start of a small consolidation wave. I don't think that's like a sign of AI winter. I keep looking for AI winter coming. I think this is kind of like a brief cold front. Yeah,
[00:07:34] NLW: it's super interesting. So I think a bunch of A bunch of stuff here.
[00:07:38] NLW: One is, I think, to both of your points, there, in some ways, there, there had already been this very clear demarcation between these two sides where, like, the GPU pores, to use the terminology, like, just weren't trying to compete on the same level, right? You know, the vast majority of people who have started something over the last year, year and a half, call it, were racing in a different direction.
[00:07:59] NLW: They're trying to find some edge somewhere else. They're trying to build something different. If they're, if they're really trying to innovate, it's in different areas. And so it's really just this very small handful of companies that are in this like very, you know, it's like the coheres and jaspers of the world that like this sort of, you know, that are that are just sort of a little bit less resourced than, you know, than the other set that I think that this potentially even applies to, you know, everyone else that could clearly demarcate it into these two, two sides.
[00:08:26] NLW: And there's only a small handful kind of sitting uncomfortably in the middle, perhaps. Let's, let's come back to the idea of, of the sort of AI winter or, you know, a cold front or anything like that. So this is something that I, I spent a lot of time kind of thinking about and noticing. And my perception is that The vast majority of the folks who are trying to call for sort of, you know, a trough of disillusionment or, you know, a shifting of the phase to that are people who either, A, just don't like AI for some other reason there's plenty of that, you know, people who are saying, You Look, they're doing way worse than they ever thought.
[00:09:03] NLW: You know, there's a lot of sort of confirmation bias kind of thing going on. Or two, media that just needs a different narrative, right? Because they're sort of sick of, you know, telling the same story. Same thing happened last summer, when every every outlet jumped on the chat GPT at its first down month story to try to really like kind of hammer this idea that that the hype was too much.
[00:09:24] NLW: Meanwhile, you have, you know, just ridiculous levels of investment from enterprises, you know, coming in. You have, you know, huge, huge volumes of, you know, individual behavior change happening. But I do think that there's nothing incoherent sort of to your point, Swyx, about that and the consolidation period.
[00:09:42] NLW: Like, you know, if you look right now, for example, there are, I don't know, probably 25 or 30 credible, like, build your own chatbot. platforms that, you know, a lot of which have, you know, raised funding. There's no universe in which all of those are successful across, you know, even with a, even, even with a total addressable market of every enterprise in the world, you know, you're just inevitably going to see some amount of consolidation.
[00:10:08] NLW: Same with, you know, image generators. There are, if you look at A16Z's top 50 consumer AI apps, just based on, you know, web traffic or whatever, they're still like I don't know, a half. Dozen or 10 or something, like, some ridiculous number of like, basically things like Midjourney or Dolly three. And it just seems impossible that we're gonna have that many, you know, ultimately as, as, as sort of, you know, going, going concerned.
[00:10:33] NLW: So, I don't know. I, I, I think that the, there will be inevitable consolidation 'cause you know. It's, it's also what kind of like venture rounds are supposed to do. You're not, not everyone who gets a seed round is supposed to get to series A and not everyone who gets a series A is supposed to get to series B.
[00:10:46] NLW: That's sort of the natural process. I think it will be tempting for a lot of people to try to infer from that something about AI not being as sort of big or as as sort of relevant as, as it was hyped up to be. But I, I kind of think that's the wrong conclusion to come to.
[00:11:02] Alessio: I I would say the experimentation.
[00:11:04] Alessio: Surface is a little smaller for image generation. So if you go back maybe six, nine months, most people will tell you, why would you build a coding assistant when like Copilot and GitHub are just going to win everything because they have the data and they have all the stuff. If you fast forward today, A lot of people use Cursor everybody was excited about the Devin release on Twitter.
[00:11:26] Alessio: There are a lot of different ways of attacking the market that are not completion of code in the IDE. And even Cursors, like they evolved beyond single line to like chat, to do multi line edits and, and all that stuff. Image generation, I would say, yeah, as a, just as from what I've seen, like maybe the product innovation has slowed down at the UX level and people are improving the models.
[00:11:50] Alessio: So the race is like, how do I make better images? It's not like, how do I make the user interact with the generation process better? And that gets tough, you know? It's hard to like really differentiate yourselves. So yeah, that's kind of how I look at it. And when we think about multimodality, maybe the reason why people got so excited about Sora is like, oh, this is like a completely It's not a better image model.
[00:12:13] Alessio: This is like a completely different thing, you know? And I think the creative mind It's always looking for something that impacts the viewer in a different way, you know, like they really want something different versus the developer mind. It's like, Oh, I, I just, I have this like very annoying thing I want better.
[00:12:32] Alessio: I have this like very specific use cases that I want to go after. So it's just different. And that's why you see a lot more companies in image generation. But I agree with you that. If you fast forward there, there's not going to be 10 of them, you know, it's probably going to be one or
[00:12:46] swyx: two. Yeah, I mean, to me, that's why I call it a war.
[00:12:49] swyx: Like, individually, all these companies can make a story that kind of makes sense, but collectively, they cannot all be true. Therefore, they all, there is some kind of fight over limited resources here. Yeah, so
[00:12:59] NLW: it's interesting. We wandered very naturally into sort of another one of these wars, which is the multimodality kind of idea, which is, you know, basically a question of whether it's going to be these sort of big everything models that end up winning or whether, you know, you're going to have really specific things, you know, like something, you know, Dolly 3 inside of sort of OpenAI's larger models versus, you know, a mid journey or something like that.
[00:13:24] NLW: And at first, you know, I was kind of thinking like, For most of the last, call it six months or whatever, it feels pretty definitively both and in some ways, you know, and that you're, you're seeing just like great innovation on sort of the everything models, but you're also seeing lots and lots happen at sort of the level of kind of individual use cases.
[00:13:45] Sora
[00:13:45] NLW: But then Sora comes along and just like obliterates what I think anyone thought you know, where we were when it comes to video generation. So how are you guys thinking about this particular battle or war at the moment?
[00:13:59] swyx: Yeah, this was definitely a both and story, and Sora tipped things one way for me, in terms of scale being all you need.
[00:14:08] swyx: And the benefit, I think, of having multiple models being developed under one roof. I think a lot of people aren't aware that Sora was developed in a similar fashion to Dolly 3. And Dolly3 had a very interesting paper out where they talked about how they sort of bootstrapped their synthetic data based on GPT 4 vision and GPT 4.
[00:14:31] swyx: And, and it was just all, like, really interesting, like, if you work on one modality, it enables you to work on other modalities, and all that is more, is, is more interesting. I think it's beneficial if it's all in the same house, whereas the individual startups who don't, who sort of carve out a single modality and work on that, definitely won't have the state of the art stuff on helping them out on synthetic data.
[00:14:52] swyx: So I do think like, The balance is tilted a little bit towards the God model companies, which is challenging for the, for the, for the the sort of dedicated modality companies. But everyone's carving out different niches. You know, like we just interviewed Suno ai, the sort of music model company, and, you know, I don't see opening AI pursuing music anytime soon.
[00:15:12] Suno
[00:15:12] swyx: Yeah,
[00:15:13] NLW: Suno's been phenomenal to play with. Suno has done that rare thing where, which I think a number of different AI product categories have done, where people who don't consider themselves particularly interested in doing the thing that the AI enables find themselves doing a lot more of that thing, right?
[00:15:29] NLW: Like, it'd be one thing if Just musicians were excited about Suno and using it but what you're seeing is tons of people who just like music all of a sudden like playing around with it and finding themselves kind of down that rabbit hole, which I think is kind of like the highest compliment that you can give one of these startups at the
[00:15:45] swyx: early days of it.
[00:15:46] swyx: Yeah, I, you know, I, I asked them directly, you know, in the interview about whether they consider themselves mid journey for music. And he had a more sort of nuanced response there, but I think that probably the business model is going to be very similar because he's focused on the B2C element of that. So yeah, I mean, you know, just to, just to tie back to the question about, you know, You know, large multi modality companies versus small dedicated modality companies.
[00:16:10] swyx: Yeah, highly recommend people to read the Sora blog posts and then read through to the Dali blog posts because they, they strongly correlated themselves with the same synthetic data bootstrapping methods as Dali. And I think once you make those connections, you're like, oh, like it, it, it is beneficial to have multiple state of the art models in house that all help each other.
[00:16:28] swyx: And these, this, that's the one thing that a dedicated modality company cannot do.
[00:16:34] The GPT-4 Class Landscape
[00:16:34] NLW: So I, I wanna jump, I wanna kind of build off that and, and move into the sort of like updated GPT-4 class landscape. 'cause that's obviously been another big change over the last couple months. But for the sake of completeness, is there anything that's worth touching on with with sort of the quality?
[00:16:46] NLW: Quality data or sort of a rag ops wars just in terms of, you know, anything that's changed, I guess, for you fundamentally in the last couple of months about where those things stand.
[00:16:55] swyx: So I think we're going to talk about rag for the Gemini and Clouds discussion later. And so maybe briefly discuss the data piece.
[00:17:03] Data War: Reddit x Google
[00:17:03] swyx: I think maybe the only new thing was this Reddit deal with Google for like a 60 million dollar deal just ahead of their IPO, very conveniently turning Reddit into a AI data company. Also, very, very interestingly, a non exclusive deal, meaning that Reddit can resell that data to someone else. And it probably does become table stakes.
[00:17:23] swyx: A lot of people don't know, but a lot of the web text dataset that originally started for GPT 1, 2, and 3 was actually scraped from GitHub. from Reddit at least the sort of vote scores. And I think, I think that's a, that's a very valuable piece of information. So like, yeah, I think people are figuring out how to pay for data.
[00:17:40] swyx: People are suing each other over data. This, this, this war is, you know, definitely very, very much heating up. And I don't think, I don't see it getting any less intense. I, you know, next to GPUs, data is going to be the most expensive thing in, in a model stack company. And. You know, a lot of people are resorting to synthetic versions of it, which may or may not be kosher based on how far along or how commercially blessed the, the forms of creating that synthetic data are.
[00:18:11] swyx: I don't know if Alessio, you have any other interactions with like Data source companies, but that's my two cents.
[00:18:17] Alessio: Yeah yeah, I actually saw Quentin Anthony from Luther. ai at GTC this week. He's also been working on this. I saw Technium. He's also been working on the data side. I think especially in open source, people are like, okay, if everybody is putting the gates up, so to speak, to the data we need to make it easier for people that don't have 50 million a year to get access to good data sets.
[00:18:38] Alessio: And Jensen, at his keynote, he did talk about synthetic data a little bit. So I think that's something that we'll definitely hear more and more of in the enterprise, which never bodes well, because then all the, all the people with the data are like, Oh, the enterprises want to pay now? Let me, let me put a pay here stripe link so that they can give me 50 million.
[00:18:57] Alessio: But it worked for Reddit. I think the stock is up. 40 percent today after opening. So yeah, I don't know if it's all about the Google deal, but it's obviously Reddit has been one of those companies where, hey, you got all this like great community, but like, how are you going to make money? And like, they try to sell the avatars.
[00:19:15] Alessio: I don't know if that it's a great business for them. The, the data part sounds as an investor, you know, the data part sounds a lot more interesting than, than consumer
[00:19:25] swyx: cosmetics. Yeah, so I think, you know there's more questions around data you know, I think a lot of people are talking about the interview that Mira Murady did with the Wall Street Journal, where she, like, just basically had no, had no good answer for where they got the data for Sora.
[00:19:39] swyx: I, I think this is where, you know, there's, it's in nobody's interest to be transparent about data, and it's, it's kind of sad for the state of ML and the state of AI research but it is what it is. We, we have to figure this out as a society, just like we did for music and music sharing. You know, in, in sort of the Napster to Spotify transition, and that might take us a decade.
[00:19:59] swyx: Yeah, I
[00:20:00] NLW: do. I, I agree. I think, I think that you're right to identify it, not just as that sort of technical problem, but as one where society has to have a debate with itself. Because I think that there's, if you rationally within it, there's Great kind of points on all side, not to be the sort of, you know, person who sits in the middle constantly, but it's why I think a lot of these legal decisions are going to be really important because, you know, the job of judges is to listen to all this stuff and try to come to things and then have other judges disagree.
[00:20:24] NLW: And, you know, and have the rest of us all debate at the same time. By the way, as a total aside, I feel like the synthetic data right now is like eggs in the 80s and 90s. Like, whether they're good for you or bad for you, like, you know, we, we get one study that's like synthetic data, you know, there's model collapse.
[00:20:42] NLW: And then we have like a hint that llama, you know, to the most high performance version of it, which was one they didn't release was trained on synthetic data. So maybe it's good. It's like, I just feel like every, every other week I'm seeing something sort of different about whether it's a good or bad for, for these models.
[00:20:56] swyx: Yeah. The branding of this is pretty poor. I would kind of tell people to think about it like cholesterol. There's good cholesterol, bad cholesterol. And you can have, you know, good amounts of both. But at this point, it is absolutely without a doubt that most large models from here on out will all be trained as some kind of synthetic data and that is not a bad thing.
[00:21:16] swyx: There are ways in which you can do it poorly. Whether it's commercial, you know, in terms of commercial sourcing or in terms of the model performance. But it's without a doubt that good synthetic data is going to help your model. And this is just a question of like where to obtain it and what kinds of synthetic data are valuable.
[00:21:36] swyx: You know, if even like alpha geometry, you know, was, was a really good example from like earlier this year.
[00:21:42] NLW: If you're using the cholesterol analogy, then my, then my egg thing can't be that far off. Let's talk about the sort of the state of the art and the, and the GPT 4 class landscape and how that's changed.
[00:21:53] Gemini 1.5 vs Claude 3
[00:21:53] NLW: Cause obviously, you know, sort of the, the two big things or a couple of the big things that have happened. Since we last talked, we're one, you know, Gemini first announcing that a model was coming and then finally it arriving, and then very soon after a sort of a different model arriving from Gemini and and Cloud three.
[00:22:11] NLW: So I guess, you know, I'm not sure exactly where the right place to start with this conversation is, but, you know, maybe very broadly speaking which of these do you think have made a bigger impact? Thank you.
[00:22:20] Alessio: Probably the one you can use, right? So, Cloud. Well, I'm sure Gemini is going to be great once they let me in, but so far I haven't been able to.
[00:22:29] Alessio: I use, so I have this small podcaster thing that I built for our podcast, which does chapters creation, like named entity recognition, summarization, and all of that. Cloud Tree is, Better than GPT 4. Cloud2 was unusable. So I use GPT 4 for everything. And then when Opus came out, I tried them again side by side and I posted it on, on Twitter as well.
[00:22:53] Alessio: Cloud is better. It's very good, you know, it's much better, it seems to me, it's much better than GPT 4 at doing writing that is more, you know, I don't know, it just got good vibes, you know, like the GPT 4 text, you can tell it's like GPT 4, you know, it's like, it always uses certain types of words and phrases and, you know, maybe it's just me because I've now done it for, you know, So, I've read like 75, 80 generations of these things next to each other.
[00:23:21] Alessio: Clutter is really good. I know everybody is freaking out on twitter about it, my only experience of this is much better has been on the podcast use case. But I know that, you know, Quran from from News Research is a very big opus pro, pro opus person. So, I think that's also It's great to have people that actually care about other models.
[00:23:40] Alessio: You know, I think so far to a lot of people, maybe Entropic has been the sibling in the corner, you know, it's like Cloud releases a new model and then OpenAI releases Sora and like, you know, there are like all these different things, but yeah, the new models are good. It's interesting.
[00:23:55] NLW: My my perception is definitely that just, just observationally, Cloud 3 is certainly the first thing that I've seen where lots of people.
[00:24:06] NLW: They're, no one's debating evals or anything like that. They're talking about the specific use cases that they have, that they used to use chat GPT for every day, you know, day in, day out, that they've now just switched over. And that has, I think, shifted a lot of the sort of like vibe and sentiment in the space too.
[00:24:26] NLW: And I don't necessarily think that it's sort of a A like full you know, sort of full knock. Let's put it this way. I think it's less bad for open AI than it is good for anthropic. I think that because GPT 5 isn't there, people are not quite willing to sort of like, you know get overly critical of, of open AI, except in so far as they're wondering where GPT 5 is.
[00:24:46] NLW: But I do think that it makes, Anthropic look way more credible as a, as a, as a player, as a, you know, as a credible sort of player, you know, as opposed to to, to where they were.
[00:24:57] Alessio: Yeah. And I would say the benchmarks veil is probably getting lifted this year. I think last year. People were like, okay, this is better than this on this benchmark, blah, blah, blah, because maybe they did not have a lot of use cases that they did frequently.
[00:25:11] Alessio: So it's hard to like compare yourself. So you, you defer to the benchmarks. I think now as we go into 2024, a lot of people have started to use these models from, you know, from very sophisticated things that they run in production to some utility that they have on their own. Now they can just run them side by side.
[00:25:29] Alessio: And it's like, Hey, I don't care that like. The MMLU score of Opus is like slightly lower than GPT 4. It just works for me, you know, and I think that's the same way that traditional software has been used by people, right? Like you just strive for yourself and like, which one does it work, works best for you?
[00:25:48] Alessio: Like nobody looks at benchmarks outside of like sales white papers, you know? And I think it's great that we're going more in that direction. We have a episode with Adapt coming out this weekend. I'll and some of their model releases, they specifically say, We do not care about benchmarks, so we didn't put them in, you know, because we, we don't want to look good on them.
[00:26:06] Alessio: We just want the product to work. And I think more and more people will, will
[00:26:09] swyx: go that way. Yeah. I I would say like, it does take the wind out of the sails for GPT 5, which I know where, you know, Curious about later on. I think anytime you put out a new state of the art model, you have to break through in some way.
[00:26:21] swyx: And what Claude and Gemini have done is effectively take away any advantage to saying that you have a million token context window. Now everyone's just going to be like, Oh, okay. Now you just match the other two guys. And so that puts An insane amount of pressure on what gpt5 is going to be because it's just going to have like the only option it has now because all the other models are multimodal all the other models are long context all the other models have perfect recall gpt5 has to match everything and do more to to not be a flop
[00:26:58] AI Breakdown Part 2
[00:26:58] NLW: hello friends back again with part two if you haven't heard part one of this conversation i suggest you go check it out but to be honest they are kind of actually separable In this conversation, we get into a topic that I think Alessio and Swyx are very well positioned to discuss, which is what developers care about right now, what people are trying to build around.
[00:27:16] NLW: I honestly think that one of the best ways to see the future in an industry like AI is to try to dig deep on what developers and entrepreneurs are attracted to build, even if it hasn't made it to the news pages yet. So consider this your preview of six months from now, and let's dive in. Let's bring it to the GPT 5 conversation.
[00:27:33] Next Frontiers: Llama 3, GPT-5, Gemini 2, Claude 4
[00:27:33] NLW: I mean, so, so I think that that's a great sort of assessment of just how the stakes have been raised, you know is your, I mean, so I guess maybe, maybe I'll, I'll frame this less as a question, just sort of something that, that I, that I've been watching right now, the only thing that makes sense to me with how.
[00:27:50] NLW: Fundamentally unbothered and unstressed OpenAI seems about everything is that they're sitting on something that does meet all that criteria, right? Because, I mean, even in the Lex Friedman interview that, that Altman recently did, you know, he's talking about other things coming out first. He's talking about, he's just like, he, listen, he, he's good and he could play nonchalant, you know, if he wanted to.
[00:28:13] NLW: So I don't want to read too much into it, but. You know, they've had so long to work on this, like unless that we are like really meaningfully running up against some constraint, it just feels like, you know, there's going to be some massive increase, but I don't know. What do you guys think?
[00:28:28] swyx: Hard to speculate.
[00:28:29] swyx: You know, at this point, they're, they're pretty good at PR and they're not going to tell you anything that they don't want to. And he can tell you one thing and change their minds the next day. So it's, it's, it's really, you know, I've always said that model version numbers are just marketing exercises, like they have something and it's always improving and at some point you just cut it and decide to call it GPT 5.
[00:28:50] swyx: And it's more just about defining an arbitrary level at which they're ready and it's up to them on what ready means. We definitely did see some leaks on GPT 4. 5, as I think a lot of people reported and I'm not sure if you covered it. So it seems like there might be an intermediate release. But I did feel, coming out of the Lex Friedman interview, that GPT 5 was nowhere near.
[00:29:11] swyx: And you know, it was kind of a sharp contrast to Sam talking at Davos in February, saying that, you know, it was his top priority. So I find it hard to square. And honestly, like, there's also no point Reading too much tea leaves into what any one person says about something that hasn't happened yet or has a decision that hasn't been taken yet.
[00:29:31] swyx: Yeah, that's, that's my 2 cents about it. Like, calm down, let's just build .
[00:29:35] Alessio: Yeah. The, the February rumor was that they were gonna work on AI agents, so I don't know, maybe they're like, yeah,
[00:29:41] swyx: they had two agent two, I think two agent projects, right? One desktop agent and one sort of more general yeah, sort of GPTs like agent and then Andre left, so he was supposed to be the guy on that.
[00:29:52] swyx: What did Andre see? What did he see? I don't know. What did he see?
[00:29:56] Alessio: I don't know. But again, it's just like the rumors are always floating around, you know but I think like, this is, you know, we're not going to get to the end of the year without Jupyter you know, that's definitely happening. I think the biggest question is like, are Anthropic and Google.
[00:30:13] Alessio: Increasing the pace, you know, like it's the, it's the cloud four coming out like in 12 months, like nine months. What's the, what's the deal? Same with Gemini. They went from like one to 1. 5 in like five days or something. So when's Gemini 2 coming out, you know, is that going to be soon? I don't know.
[00:30:31] Alessio: There, there are a lot of, speculations, but the good thing is that now you can see a world in which OpenAI doesn't rule everything. You know, so that, that's the best, that's the best news that everybody got, I would say.
[00:30:43] swyx: Yeah, and Mistral Large also dropped in the last month. And, you know, not as, not quite GPT 4 class, but very good from a new startup.
[00:30:52] swyx: So yeah, we, we have now slowly changed in landscape, you know. In my January recap, I was complaining that nothing's changed in the landscape for a long time. But now we do exist in a world, sort of a multipolar world where Cloud and Gemini are legitimate challengers to GPT 4 and hopefully more will emerge as well hopefully from meta.
[00:31:11] Open Source Models - Mistral, Grok
[00:31:11] NLW: So speak, let's actually talk about sort of the open source side of this for a minute. So Mistral Large, notable because it's, it's not available open source in the same way that other things are, although I think my perception is that the community has largely given them Like the community largely recognizes that they want them to keep building open source stuff and they have to find some way to fund themselves that they're going to do that.
[00:31:27] NLW: And so they kind of understand that there's like, they got to figure out how to eat, but we've got, so, you know, there there's Mistral, there's, I guess, Grok now, which is, you know, Grok one is from, from October is, is open
[00:31:38] swyx: sourced at, yeah. Yeah, sorry, I thought you thought you meant Grok the chip company.
[00:31:41] swyx: No, no, no, yeah, you mean Twitter Grok.
[00:31:43] NLW: Although Grok the chip company, I think is even more interesting in some ways, but and then there's the, you know, obviously Llama3 is the one that sort of everyone's wondering about too. And, you know, my, my sense of that, the little bit that, you know, Zuckerberg was talking about Llama 3 earlier this year, suggested that, at least from an ambition standpoint, he was not thinking about how do I make sure that, you know, meta content, you know, keeps, keeps the open source thrown, you know, vis a vis Mistral.
[00:32:09] NLW: He was thinking about how you go after, you know, how, how he, you know, releases a thing that's, you know, every bit as good as whatever OpenAI is on at that point.
[00:32:16] Alessio: Yeah. From what I heard in the hallways at, at GDC, Llama 3, the, the biggest model will be, you 260 to 300 billion parameters, so that that's quite large.
[00:32:26] Alessio: That's not an open source model. You know, you cannot give people a 300 billion parameters model and ask them to run it. You know, it's very compute intensive. So I think it is, it
[00:32:35] swyx: can be open source. It's just, it's going to be difficult to run, but that's a separate question.
[00:32:39] Alessio: It's more like, as you think about what they're doing it for, you know, it's not like empowering the person running.
[00:32:45] Alessio: llama. On, on their laptop, it's like, oh, you can actually now use this to go after open AI, to go after Anthropic, to go after some of these companies at like the middle complexity level, so to speak. Yeah. So obviously, you know, we estimate Gentala on the podcast, they're doing a lot here, they're making PyTorch better.
[00:33:03] Alessio: You know, they want to, that's kind of like maybe a little bit of a shorted. Adam Bedia, in a way, trying to get some of the CUDA dominance out of it. Yeah, no, it's great. The, I love the duck destroying a lot of monopolies arc. You know, it's, it's been very entertaining. Let's bridge
[00:33:18] NLW: into the sort of big tech side of this, because this is obviously like, so I think actually when I did my episode, this was one of the I added this as one of as an additional war that, that's something that I'm paying attention to.
[00:33:29] NLW: So we've got Microsoft's moves with inflection, which I think pretend, potentially are being read as A shift vis a vis the relationship with OpenAI, which also the sort of Mistral large relationship seems to reinforce as well. We have Apple potentially entering the race, finally, you know, giving up Project Titan and and, and kind of trying to spend more effort on this.
[00:33:50] NLW: Although, Counterpoint, we also have them talking about it, or there being reports of a deal with Google, which, you know, is interesting to sort of see what their strategy there is. And then, you know, Meta's been largely quiet. We kind of just talked about the main piece, but, you know, there's, and then there's spoilers like Elon.
[00:34:07] NLW: I mean, you know, what, what of those things has sort of been most interesting to you guys as you think about what's going to shake out for the rest of this
[00:34:13] Apple MM1
[00:34:13] swyx: year? I'll take a crack. So the reason we don't have a fifth war for the Big Tech Wars is that's one of those things where I just feel like we don't cover differently from other media channels, I guess.
[00:34:26] swyx: Sure, yeah. In our anti interestness, we actually say, like, we try not to cover the Big Tech Game of Thrones, or it's proxied through Twitter. You know, all the other four wars anyway, so there's just a lot of overlap. Yeah, I think absolutely, personally, the most interesting one is Apple entering the race.
[00:34:41] swyx: They actually released, they announced their first large language model that they trained themselves. It's like a 30 billion multimodal model. People weren't that impressed, but it was like the first time that Apple has kind of showcased that, yeah, we're training large models in house as well. Of course, like, they might be doing this deal with Google.
[00:34:57] swyx: I don't know. It sounds very sort of rumor y to me. And it's probably, if it's on device, it's going to be a smaller model. So something like a Jemma. It's going to be smarter autocomplete. I don't know what to say. I'm still here dealing with, like, Siri, which hasn't, probably hasn't been updated since God knows when it was introduced.
[00:35:16] swyx: It's horrible. I, you know, it, it, it makes me so angry. So I, I, one, as an Apple customer and user, I, I'm just hoping for better AI on Apple itself. But two, they are the gold standard when it comes to local devices, personal compute and, and trust, like you, you trust them with your data. And. I think that's what a lot of people are looking for in AI, that they have, they love the benefits of AI, they don't love the downsides, which is that you have to send all your data to some cloud somewhere.
[00:35:45] swyx: And some of this data that we're going to feed AI is just the most personal data there is. So Apple being like one of the most trusted personal data companies, I think it's very important that they enter the AI race, and I hope to see more out of them.
[00:35:58] Alessio: To me, the, the biggest question with the Google deal is like, who's paying who?
[00:36:03] Alessio: Because for the browsers, Google pays Apple like 18, 20 billion every year to be the default browser. Is Google going to pay you to have Gemini or is Apple paying Google to have Gemini? I think that's, that's like what I'm most interested to figure out because with the browsers, it's like, it's the entry point to the thing.
[00:36:21] Alessio: So it's really valuable to be the default. That's why Google pays. But I wonder if like the perception in AI is going to be like, Hey. You just have to have a good local model on my phone to be worth me purchasing your device. And that was, that's kind of drive Apple to be the one buying the model. But then, like Shawn said, they're doing the MM1 themselves.
[00:36:40] Alessio: So are they saying we do models, but they're not as good as the Google ones? I don't know. The whole thing is, it's really confusing, but. It makes for great meme material on on Twitter.
[00:36:51] swyx: Yeah, I mean, I think, like, they are possibly more than OpenAI and Microsoft and Amazon. They are the most full stack company there is in computing, and so, like, they own the chips, man.
[00:37:05] swyx: Like, they manufacture everything so if, if, if there was a company that could do that. You know, seriously challenge the other AI players. It would be Apple. And it's, I don't think it's as hard as self driving. So like maybe they've, they've just been investing in the wrong thing this whole time. We'll see.
[00:37:21] swyx: Wall Street certainly thinks
[00:37:22] NLW: so. Wall Street loved that move, man. There's a big, a big sigh of relief. Well, let's, let's move away from, from sort of the big stuff. I mean, the, I think to both of your points, it's going to.
[00:37:33] Meta's $800b AI rebrand
[00:37:33] NLW: Can I, can
[00:37:34] swyx: I, can I, can I jump on factoid about this, this Wall Street thing? I went and looked at when Meta went from being a VR company to an AI company.
[00:37:44] swyx: And I think the stock I'm trying to look up the details now. The stock has gone up 187% since Lamo one. Yeah. Which is $830 billion in market value created in the past year. . Yeah. Yeah.
[00:37:57] NLW: It's, it's, it's like, remember if you guys haven't Yeah. If you haven't seen the chart, it's actually like remarkable.
[00:38:02] NLW: If you draw a little
[00:38:03] swyx: arrow on it, it's like, no, we're an AI company now and forget the VR thing.
[00:38:10] NLW: It's it, it is an interesting, no, it's, I, I think, alessio, you called it sort of like Zuck's Disruptor Arc or whatever. He, he really does. He is in the midst of a, of a total, you know, I don't know if it's a redemption arc or it's just, it's something different where, you know, he, he's sort of the spoiler.
[00:38:25] NLW: Like people loved him just freestyle talking about why he thought they had a better headset than Apple. But even if they didn't agree, they just loved it. He was going direct to camera and talking about it for, you know, five minutes or whatever. So that, that's a fascinating shift that I don't think anyone had on their bingo card, you know, whatever, two years ago.
[00:38:41] NLW: Yeah. Yeah,
[00:38:42] swyx: we still
[00:38:43] Alessio: didn't see and fight Elon though, so
[00:38:45] swyx: that's what I'm really looking forward to. I mean, hey, don't, don't, don't write it off, you know, maybe just these things take a while to happen. But we need to see and fight in the Coliseum. No, I think you know, in terms of like self management, life leadership, I think he has, there's a lot of lessons to learn from him.
[00:38:59] swyx: You know he might, you know, you might kind of quibble with, like, the social impact of Facebook, but just himself as a in terms of personal growth and, and, you know, Per perseverance through like a lot of change and you know, everyone throwing stuff his way. I think there's a lot to say about like, to learn from, from Zuck, which is crazy 'cause he's my age.
[00:39:18] swyx: Yeah. Right.
[00:39:20] AI Engineer landscape - from baby AGIs to vertical Agents
[00:39:20] NLW: Awesome. Well, so, so one of the big things that I think you guys have, you know, distinct and, and unique insight into being where you are and what you work on is. You know, what developers are getting really excited about right now. And by that, I mean, on the one hand, certainly, you know, like startups who are actually kind of formalized and formed to startups, but also, you know, just in terms of like what people are spending their nights and weekends on what they're, you know, coming to hackathons to do.
[00:39:45] NLW: And, you know, I think it's a, it's a, it's, it's such a fascinating indicator for, for where things are headed. Like if you zoom back a year, right now was right when everyone was getting so, so excited about. AI agent stuff, right? Auto, GPT and baby a GI. And these things were like, if you dropped anything on YouTube about those, like instantly tens of thousands of views.
[00:40:07] NLW: I know because I had like a 50,000 view video, like the second day that I was doing the show on YouTube, you know, because I was talking about auto GPT. And so anyways, you know, obviously that's sort of not totally come to fruition yet, but what are some of the trends in what you guys are seeing in terms of people's, people's interest and, and, and what people are building?
[00:40:24] Alessio: I can start maybe with the agents part and then I know Shawn is doing a diffusion meetup tonight. There's a lot of, a lot of different things. The, the agent wave has been the most interesting kind of like dream to reality arc. So out of GPT, I think they went, From zero to like 125, 000 GitHub stars in six weeks, and then one year later, they have 150, 000 stars.
[00:40:49] Alessio: So there's kind of been a big plateau. I mean, you might say there are just not that many people that can start it. You know, everybody already started it. But the promise of, hey, I'll just give you a goal, and you do it. I think it's like, amazing to get people's imagination going. You know, they're like, oh, wow, this This is awesome.
[00:41:08] Alessio: Everybody, everybody can try this to do anything. But then as technologists, you're like, well, that's, that's just like not possible, you know, we would have like solved everything. And I think it takes a little bit to go from the promise and the hope that people show you to then try it yourself and going back to say, okay, this is not really working for me.
[00:41:28] Alessio: And David Wong from Adept, you know, they in our episode, he specifically said. We don't want to do a bottom up product. You know, we don't want something that everybody can just use and try because it's really hard to get it to be reliable. So we're seeing a lot of companies doing vertical agents that are narrow for a specific domain, and they're very good at something.
[00:41:49] Alessio: Mike Conover, who was at Databricks before, is also a friend of Latentspace. He's doing this new company called BrightWave doing AI agents for financial research, and that's it, you know, and they're doing very well. There are other companies doing it in security, doing it in compliance, doing it in legal.
[00:42:08] Alessio: All of these things that like, people, nobody just wakes up and say, Oh, I cannot wait to go on AutoGPD and ask it to do a compliance review of my thing. You know, just not what inspires people. So I think the gap on the developer side has been the more bottom sub hacker mentality is trying to build this like very Generic agents that can do a lot of open ended tasks.
[00:42:30] Alessio: And then the more business side of things is like, Hey, If I want to raise my next round, I can not just like sit around the mess, mess around with like super generic stuff. I need to find a use case that really works. And I think that that is worth for, for a lot of folks in parallel, you have a lot of companies doing evals.
[00:42:47] Alessio: There are dozens of them that just want to help you measure how good your models are doing. Again, if you build evals, you need to also have a restrained surface area to actually figure out whether or not it's good, right? Because you cannot eval anything on everything under the sun. So that's another category where I've seen from the startup pitches that I've seen, there's a lot of interest in, in the enterprise.
[00:43:11] Alessio: It's just like really. Fragmented because the production use cases are just coming like now, you know, there are not a lot of long established ones to, to test against. And so does it, that's kind of on the virtual agents and then the robotic side it's probably been the thing that surprised me the most at NVIDIA GTC, the amount of robots that were there that were just like robots everywhere.
[00:43:33] Alessio: Like, both in the keynote and then on the show floor, you would have Boston Dynamics dogs running around. There was, like, this, like fox robot that had, like, a virtual face that, like, talked to you and, like, moved in real time. There were industrial robots. NVIDIA did a big push on their own Omniverse thing, which is, like, this Digital twin of whatever environments you're in that you can use to train the robots agents.
[00:43:57] Alessio: So that kind of takes people back to the reinforcement learning days, but yeah, agents, people want them, you know, people want them. I give a talk about the, the rise of the full stack employees and kind of this future, the same way full stack engineers kind of work across the stack. In the future, every employee is going to interact with every part of the organization through agents and AI enabled tooling.
[00:44:17] Alessio: This is happening. It just needs to be a lot more narrow than maybe the first approach that we took, which is just put a string in AutoGPT and pray. But yeah, there's a lot of super interesting stuff going on.
[00:44:27] swyx: Yeah. Well, he Let's recover a lot of stuff there. I'll separate the robotics piece because I feel like that's so different from the software world.
[00:44:34] swyx: But yeah, we do talk to a lot of engineers and you know, that this is our sort of bread and butter. And I do agree that vertical agents have worked out a lot better than the horizontal ones. I think all You know, the point I'll make here is just the reason AutoGPT and maybe AGI, you know, it's in the name, like they were promising AGI.
[00:44:53] swyx: But I think people are discovering that you cannot engineer your way to AGI. It has to be done at the model level and all these engineering, prompt engineering hacks on top of it weren't really going to get us there in a meaningful way without much further, you know, improvements in the models. I would say, I'll go so far as to say, even Devin, which is, I would, I think the most advanced agent that we've ever seen, still requires a lot of engineering and still probably falls apart a lot in terms of, like, practical usage.
[00:45:22] swyx: Or it's just, Way too slow and expensive for, you know, what it's, what it's promised compared to the video. So yeah, that's, that's what, that's what happened with agents from, from last year. But I, I do, I do see, like, vertical agents being very popular and, and sometimes you, like, I think the word agent might even be overused sometimes.
[00:45:38] swyx: Like, people don't really care whether or not you call it an AI agent, right? Like, does it replace boring menial tasks that I do That I might hire a human to do, or that the human who is hired to do it, like, actually doesn't really want to do. And I think there's absolutely ways in sort of a vertical context that you can actually go after very routine tasks that can be scaled out to a lot of, you know, AI assistants.
[00:46:01] swyx: So, so yeah, I mean, and I would, I would sort of basically plus one what let's just sit there. I think it's, it's very, very promising and I think more people should work on it, not less. Like there's not enough people. Like, we, like, this should be the, the, the main thrust of the AI engineer is to look out, look for use cases and, and go to a production with them instead of just always working on some AGI promising thing that never arrives.
[00:46:21] swyx: I,
[00:46:22] NLW: I, I can only add that so I've been fiercely making tutorials behind the scenes around basically everything you can imagine with AI. We've probably done, we've done about 300 tutorials over the last couple of months. And the verticalized anything, right, like this is a solution for your particular job or role, even if it's way less interesting or kind of sexy, it's like so radically more useful to people in terms of intersecting with how, like those are the ways that people are actually.
[00:46:50] NLW: Adopting AI in a lot of cases is just a, a, a thing that I do over and over again. By the way, I think that's the same way that even the generalized models are getting adopted. You know, it's like, I use midjourney for lots of stuff, but the main thing I use it for is YouTube thumbnails every day. Like day in, day out, I will always do a YouTube thumbnail, you know, or two with, with Midjourney, right?
[00:47:09] NLW: And it's like you can, you can start to extrapolate that across a lot of things and all of a sudden, you know, a AI doesn't. It looks revolutionary because of a million small changes rather than one sort of big dramatic change. And I think that the verticalization of agents is sort of a great example of how that's
[00:47:26] swyx: going to play out too.
[00:47:28] Adept episode - Screen Multimodality
[00:47:28] swyx: So I'll have one caveat here, which is I think that Because multi modal models are now commonplace, like Cloud, Gemini, OpenAI, all very very easily multi modal, Apple's easily multi modal, all this stuff. There is a switch for agents for sort of general desktop browsing that I think people so much for joining us today, and we'll see you in the next video.
[00:48:04] swyx: Version of the the agent where they're not specifically taking in text or anything They're just watching your screen just like someone else would and and I'm piloting it by vision And you know in the the episode with David that we'll have dropped by the time that this this airs I think I think that is the promise of adept and that is a promise of what a lot of these sort of desktop agents Are and that is the more general purpose system That could be as big as the browser, the operating system, like, people really want to build that foundational piece of software in AI.
[00:48:38] swyx: And I would see, like, the potential there for desktop agents being that, that you can have sort of self driving computers. You know, don't write the horizontal piece out. I just think we took a while to get there.
[00:48:48] NLW: What else are you guys seeing that's interesting to you? I'm looking at your notes and I see a ton of categories.
[00:48:54] Top Model Research from January Recap
[00:48:54] swyx: Yeah so I'll take the next two as like as one category, which is basically alternative architectures, right? The two main things that everyone following AI kind of knows now is, one, the diffusion architecture, and two, the let's just say the, Decoder only transformer architecture that is popularized by GPT.
[00:49:12] swyx: You can read, you can look on YouTube for thousands and thousands of tutorials on each of those things. What we are talking about here is what's next, what people are researching, and what could be on the horizon that takes the place of those other two things. So first of all, we'll talk about transformer architectures and then diffusion.
[00:49:25] swyx: So transformers the, the two leading candidates are effectively RWKV and the state space models the most recent one of which is Mamba, but there's others like the Stripe, ENA, and the S four H three stuff coming out of hazy research at Stanford. And all of those are non quadratic language models that scale the promise to scale a lot better than the, the traditional transformer.
[00:49:47] swyx: That this might be too theoretical for most people right now, but it's, it's gonna be. It's gonna come out in weird ways, where, imagine if like, Right now the talk of the town is that Claude and Gemini have a million tokens of context and like whoa You can put in like, you know, two hours of video now, okay But like what if you put what if we could like throw in, you know, two hundred thousand hours of video?
[00:50:09] swyx: Like how does that change your usage of AI? What if you could throw in the entire genetic sequence of a human and like synthesize new drugs. Like, well, how does that change things? Like, we don't know because we haven't had access to this capability being so cheap before. And that's the ultimate promise of these two models.
[00:50:28] swyx: They're not there yet but we're seeing very, very good progress. RWKV and Mamba are probably the, like, the two leading examples, both of which are open source that you can try them today and and have a lot of progress there. And the, the, the main thing I'll highlight for audio e KV is that at, at the seven B level, they seem to have beat LAMA two in all benchmarks that matter at the same size for the same amount of training as an open source model.
[00:50:51] swyx: So that's exciting. You know, they're there, they're seven B now. They're not at seven tb. We don't know if it'll. And then the other thing is diffusion. Diffusions and transformers are are kind of on the collision course. The original stable diffusion already used transformers in in parts of its architecture.
[00:51:06] swyx: It seems that transformers are eating more and more of those layers particularly the sort of VAE layer. So that's, the Diffusion Transformer is what Sora is built on. The guy who wrote the Diffusion Transformer paper, Bill Pebbles, is, Bill Pebbles is the lead tech guy on Sora. So you'll just see a lot more Diffusion Transformer stuff going on.
[00:51:25] swyx: But there's, there's more sort of experimentation with diffusion. I'm holding a meetup actually here in San Francisco that's gonna be like the state of diffusion, which I'm pretty excited about. Stability's doing a lot of good work. And if you look at the, the architecture of how they're creating Stable Diffusion 3, Hourglass Diffusion, and the inconsistency models, or SDXL Turbo.
[00:51:45] swyx: All of these are, like, very, very interesting innovations on, like, the original idea of what Stable Diffusion was. So if you think that it is expensive to create or slow to create Stable Diffusion or an AI generated art, you are not up to date with the latest models. If you think it is hard to create text and images, you are not up to date with the latest models.
[00:52:02] swyx: And people still are kind of far behind. The last piece of which is the wildcard I always kind of hold out, which is text diffusion. So Instead of using autogenerative or autoregressive transformers, can you use text to diffuse? So you can use diffusion models to diffuse and create entire chunks of text all at once instead of token by token.
[00:52:22] swyx: And that is something that Midjourney confirmed today, because it was only rumored the past few months. But they confirmed today that they were looking into. So all those things are like very exciting new model architectures that are, Maybe something that we'll, you'll see in production two to three years from now.
[00:52:37] swyx: So the couple of the trends
[00:52:38] NLW: that I want to just get your takes on, because they're sort of something that, that seems like they're coming up are one sort of these, these wearable, you know, kind of passive AI experiences where they're absorbing a lot of what's going on around you and then, and then kind of bringing things back.
[00:52:53] NLW: And then the, the other one that I, that I wanted to see if you guys had thoughts on were sort of this next generation of chip companies. Obviously there's a huge amount of emphasis. On on hardware and silicon and, and, and different ways of doing things, but, you know, love your take on, on either or both of
[00:53:07] swyx: those.
[00:53:08] AI Wearables
[00:53:08] swyx: So for so wearables, I'm very excited about it. I want wearables on me at all times. I have two right here. To, to quantify my health. And I, you know, I'm all for them. But society is not ready for wearables, right? Like, no one's comfortable with a device on recording every single conversation we have.
[00:53:24] swyx: Even all three of us here as podcasters, we don't record everything that we say. And I think there's a social shift that needs to happen. I am an investor in TAB. They are renaming to a broader vision, but they are one of the three or four leading wearables in this space. It's sort of the AI pendants, or AI OS, or AI personal companion space.
[00:53:47] swyx: I have seen two humanes in the wild in San Francisco. I'm very, very excited to report that there are people walking around with those things on their chest and it is as goofy as it sounds. It, it absolutely is going to fail. God bless them for trying. And I've also bought a rabbit. So I'm, I'm very excited for all those things to arrive.
[00:54:06] swyx: But yeah people are very keen on hardware. I think the, the, the idea that you can have physical objects that. Embody an AI that do specific things for you is as old as, you know, the sort of Golem in sort of medieval times in terms of like how much we want our objects to be smart and do things for us.
[00:54:27] swyx: And I think it's absolutely a great play. The funny thing is people are much more willing to pay you upfront for a hardware device than they are willing to pay like an 8 a month subscription recurring for software, right? And so the interesting economics of these wearable companies is they have negative float.
[00:54:47] swyx: In the sense that people pay deposits upfront, like I paid like, I don't know, 200 bucks for the rabbit. Upfront, and I don't get it for another six months. I paid 600 for the tab, and I don't get it for another six months. And, and then, then they can take that money and, and sort of invest it in like their next, the next events or their next properties or ventures.
[00:55:06] swyx: And like, I think that's a, that's a very interesting reversal of economics from other types of AI companies that I see. And I think, yeah, just the, the, the tactile feel of an AI, I think is very promising. I, Alex, I don't know if you have other thoughts on, on the wearable stuff.
[00:55:21] Alessio: The open interpreter just announced their product four hours ago.
[00:55:25] Alessio: Yeah. Which is a, it's not really a wearable, but it's a, it's still like a physical device.
[00:55:30] swyx: It's a push to talk mic to, to a device on your, on your laptop. Right. It's a $99 push talk. Yeah.
[00:55:38] Alessio: But, but, but everybody, but again, going back to your point, it's like people want to, people are interested in spending money for like things that they can hold, you know, I don't know what that means overall for like where things are going, but making more of this AI be a physical part of your life.
[00:55:54] Alessio: I think people are interested in that, but I agree with Shawn. I mean, I've been. I talked to Avi about this, but Avi's point is like, most consumers, like, care about utility more than they care about privacy, you know, like you've seen with social media. But I also think there's a big societal reaction to AI that is, like, much more rooted than the social media one.
[00:56:16] Alessio: But we'll see. But a lot, again, a lot of work, a lot of developers, a lot of money going into it. So there's, there's bound to be experiments being run. On, on the
[00:56:25] swyx: chip side. Sorry, I'll just ship it one more thing and then we transition to the chips. The thing I'll caution people on is don't overly focus on the form factor.
[00:56:33] swyx: The form factor is a delivery mode. There will be many form factors. It doesn't matter so much as where in the data war does it sit. It actually is context acquisition. Because, and maybe a little bit of multi modality. Context, like, context is king. Like, if you have access to data that no one else has, then you will be able to create AI that no one else can create.
[00:56:54] swyx: And so what is the most personal context? It is your everyday conversation. It is as close to mapping your mental train of thought As possible without, you know, physically you writing down notes. So, so that is the promise, the ultimate goal here, which is like, personal context, it's always available on you you know, loading and seeing all that stuff.
[00:57:12] swyx: But yeah, that's the, that's the frame I want to give people that the form factors will change and there will be multiple form factors, but it's the software behind that. And in the personal context that you cannot get anywhere else, that'll win.
[00:57:24] Alessio: Yeah, so that was wearables.
[00:57:26] Groq vs Nvidia month - GPU Chip War
[00:57:26] Alessio: On the chip side, yeah, Grok was probably the biggest release.
[00:57:29] Alessio: Jonathan, well, it's not even a new release because the company, I think, was started in 2016. So it's actually quite old. But now recently captured the people's imagination with their MixedREL 500 tokens a second demo. Yeah, I think so far the battle on the GPU side has been Either you go kind of like massive chip, like the Cerebros of the world, where one chip from Cerebros is about two million dollars, you know, that's compared, obviously, you cannot compare one chip versus one chip, but h100 is like 40, 000, something like that the problem with those architectures has been They want to be very general, you know, but like they wanted to put a lot of the RAM, the SRAM on the chip.
[00:58:13] Alessio: It's much more convenient when you're using larger language models, but the models outpace the size of the chips and chips have a much longer, you know, turnaround cycle. Grok today. It's great for the current architecture. It's a lot more expensive also, as far as dollar per flop but their idea is like, hey, when you have very high concurrency, we actually were much cheaper, you know, you shouldn't just be looking at the compute power for most people, this doesn't really matter, you know, like, I think that's like the most the most interesting thing to me is like, We've now gone back with, with AI to a world where developers care about what hardware is running, which was not the case in traditional software for like, maybe 20 years since as the cloud has gotten really big.
[00:58:57] Alessio: My, my thinking is that in the next two, three years, like we're going to go back to that. We're like, people are not going to be sweating. Oh, what GPU do you have in your cloud? What do you have? It's like. Yeah, you want to run this model, we can run it at the same speed as everybody else, and then everybody will make different choices, whether they want to have higher front end capital investment, and then better utilization, some people would rather do lower investment before, and then upgrade later, there are a lot of parameters and then there's the dark horses, right, that is some of the smaller companies like Lemurian Labs, MedEx that are working on maybe not a chip alone, but also like some of the, the actual math infrastructure and the instructions on it that make them run.
[00:59:40] Alessio: There's a lot going on, but yeah, I think the, the episode with with Dylan will be interesting for, for people, but I think we also came out of it saying, Hey, everybody has pros and cons. There's no, it's different than the models where you're like, Oh, this one is definitely better for me. And I'm going to use it.
[00:59:56] Alessio: I think for most people. It's like fun Twitter memeing, you know, but it's like 99 percent of people that tweet about this stuff are never gonna buy any of these chips anyway. It's, it's really more for entertainment.
[01:00:10] swyx: No. Wow. I mean, like, this is serious business here, right? You're talking about, you know, like who, like the potential new Nvidia, if anyone can take like 1% of NVIDIA's business, they're a serious startup that you should look at.
[01:00:20] swyx: Right? So , that's, that's, that's my, well, yeah,
[01:00:23] Alessio: yeah. On matters. Well, I'm more talking about like, what, how should people think about it? You know? It's like, yeah. I think like the, the end user is not impacted as much.
[01:00:31] Disagreements
[01:00:31] Alessio: This is obviously, so
[01:00:32] swyx: I disagree. Yeah, I love disagreements because, you know, who likes a podcast where all three people always agree with each other?
[01:00:38] swyx: You will see the impact of this in the tokens per second over time. This year, I have very, very credible sources all telling me that the average tokens per second, right now, we have somewhere between 50 to 100 as like the norm for people. Average tokens per second will go to 500 to 2, 000. This year from, from a number of chip suppliers that I cannot name.
[01:00:58] swyx: So like that is, that is, that will cause a step change in the use cases. Every time you have an order of magnitude improvement in the, in the speed of something, you unlock new use cases that become fun instead of a chore. And so that's what I would caution this audience to think about, which is like, what can you do in much higher AI speed?
[01:01:17] swyx: It's not just things streaming out faster. It is things working in the background a lot more seamlessly and therefore being a lot more useful. Then previously imagined. So that would be my two cents on.
[01:01:30] Alessio: Yeah. Yeah. I mean, the, the new NVIDIA chips are also much faster. To me, that's true. When it comes to startups, it's like, are the startups pushing the performance on the incumbents or are the incumbents still leading?
[01:01:44] Alessio: And then the startups are like riding the same wave, you know? I don't have yet a good sense of that. It's like, you know, it's next year's NVIDIA release. Just gonna be better than everything that gets released this year, you know, if that's the case, it's like, okay, damn Jensen, you know, it's like the meme.
[01:02:00] Alessio: It's like, I'm gonna fight. I'm gonna fight NVIDIA. It's like, damn, Jensen got hands. He really does.
[01:02:08] Summer 2024 Predictions
[01:02:08] NLW: Well, awesome conversation, guys. I guess just just by way of wrapping up, I call it over the next three months between now and sort of the beginning of summer was one prediction that each of you has. It can be about anything. It can be a big company. It can be a startup. It can be something you have privileged information that you know, and you just won't tell us that you actually
[01:02:25] Alessio: know.
[01:02:26] Alessio: What, does it have to be something that we think it's going to be true or like something that we think? Because for me, it's like, is Sundar going to be the CEO of Google? Maybe not in three months, maybe in like six months, nine months, you know, people are like, Oh, maybe Demis is going to be the new CEO.
[01:02:41] Alessio: That was kind of like, I, I was busy like fishing some deep mind people and Google people for like a good guest for the pod. And I was like, Oh, what about. Jeff Dean, and they're like, well, Demis is really like the person that runs everything anyway, and the stuff. It's like interesting. And
[01:02:57] swyx: so I don't know.
[01:02:58] swyx: What about Sergei? Sergei Sergei could come back. I don't know. Like he's making more appearances these days.
[01:03:03] Alessio: Yeah. I don't, I I Then we can just put it as like, you know. Yeah. My, my thing is like CEO change potential, but I, again, three months is too short to make a prediction. Yeah. I
[01:03:16] NLW: think that's the, that's that's fine.
[01:03:18] NLW: The, the timescale might be off.
[01:03:22] swyx: Yeah. I mean for me, I, I think the. Progression in vertical agent companies will keep going. We just had, the other day, Klarna talking about how they replaced like 700 of their customer support agents with the AI agents. That's just the beginning, guys. Like, imagine this rolling out across most of the Fortune 500.
[01:03:43] swyx: This is, and I'm not saying this is like a utopian scenario, there will be very, very embarrassing and bad outcomes of this, where like, humans would never make this mistake, but AIs did, and like, we'll all laugh at it, or we'll be very offended by whatever, you know, bad outcome it did. So we have to be responsible and careful in the rollout, but yeah, this is, it's rolling out, you know, Alessio likes to say that this year's the year of AI in production.
[01:04:04] swyx: Let's see it, let's, let's see all these sort of vertical, full stack employees. Come out into the workforce. Love
[01:04:11] Alessio: it.
[01:04:11] NLW: All right, guys. Well, thank you so much for for sharing your your thoughts and insights here And I can't wait to do it again
[01:04:18] Thursday Nights in AI - swyx
[01:04:18] NLW: Welcome
[01:04:19] swyx: back again. It's Charlie your AI co host We're now in part two of the special weekend episode collating some of SWIX and Alessio's recent appearances If you're not active in the Latentspace Discord, you might not be aware of the many, many, many in person.
[01:04:36] swyx: Events we host gathering our listener community all over the world. You can see the Latentspace community page for how to join and subscribe to our event calendar for future meetups. We're going to share some of our recent live appearances in this next part, starting with the Thursday nights in AI meetup, a regular fixture in the SF AI scene run by Imbue and Outset Capital.
[01:04:59] swyx: Primarily, our former guest, Kanjin Q, Ali Rhoda, and Josh Albrecht. Here's Swyx.
[01:05:08] swyx: Today, for those of you who have been here before, you know the general format. So we'll do a quick fireside Q& A with Swyx. Swyx, where we're asking him the questions. Then we'll actually go to our rapid fire Q& A, where we're asking really fast, hopefully, spicy questions. And then we'll open it up to the audience for your questions.
[01:05:25] swyx: So you guys sneak around the room, submit your questions, and we'll go through as many of them as possible during that period. And then actually, Swyx brought a gift for us, which is two Latentspace t shirts. AI Engineer. AI Engineer t shirts. And those will be awarded to the Two spiciest question askers.
[01:05:44] swyx: So and I'll let Josh decide on that. So if we want to get your spiciest takes, please send them in during the event as we're talking and then also at the end. All right. With that, let's get going.
[01:05:57] NLW: Okay. Welcome, Swyx. Thank you for that
[01:06:01] swyx: intro.
[01:06:01] NLW: How does it
[01:06:01] swyx: feel to be interviewed
[01:06:03] NLW: rather than the interviewer?
[01:06:04] swyx: Weird. I don't know what to do in this chair. Yeah. Like,
[01:06:07] NLW: where should I put my hands? Yeah, exactly. You look good.
[01:06:10] swyx: You look good. And I also love asking follow up questions. And I tend to, like, sort of take over panels a lot. If you ever see me on a panel, I tend to ask the other panelists questions.
[01:06:18] swyx: Okay.
[01:06:19] NLW: So we should be ready is what you're saying. So you back.
[01:06:21] swyx: That's fine. This is like a free MBU interview, so why not? That's right. That's right. That's
[01:06:24] NLW: right.
[01:06:25] swyx: Yeah, so you interviewed Ken Jeon, the CEO you didn't interview Josh, right? No, no. So maybe tonight. Yeah. Okay. We'll see. We'll look for different questions and look for an alignment.
[01:06:35] NLW: I love it. All
[01:06:36] swyx: right. I just want to hear this story. You know, you've completely exploded LatentSpace and AI Engineer, and I know you also, before all of that, had exploded in popularity for your learning in public movement and your DevTools work. And devrelations work. So, who are you and how did you get here?
[01:06:53] swyx: Let's
[01:06:53] NLW: start with that.
[01:06:54] swyx: Quick story is, I'm Shawn, I'm from Singapore. Swyx is my initials. For those who don't know, A lot of Singaporeans are ethically Chinese, and we have Chinese names and English names. So, it's just it's just my initials. Came to col came to the US for college, and have been here for about 15 years, but most, like half of that was in finance and then the other half was, was in tech.
[01:07:13] swyx: And the, and tech is where I was most known just because I realized that I was much more aligned towards learning in public, whereas in finance, Everything's a trade secret. Everything is zero sum. Whereas in tech, like, you're allowed to come to meetups and conferences and share your learnings and share your mistakes even.
[01:07:31] swyx: And that's totally fine. You, like, open source your code. It's totally fine. And even, even better, you, like, contribute PRs to other people's code, which is even better. And I found that I thrived in that. Learning public environments and that, that kind of got me started. I was an early hire, early Draft Relations hire at Netlify and then did the same at AWS Temporal and Airbyte.
[01:07:53] swyx: And then, and so that, that's like the whole story. I can talk, talk more about like developer tooling and developer relations if, if that's something that people are interested in. But I think the, the more recent thing is AI. And I started really being interested in it mostly because It, it, the, the approximate cause of starting Leanspace was stable diffusion.
[01:08:10] swyx: When you could run a large model that could do sufficiently enough on your, on your desktop. Where I was like, okay, like, this is, Something qualitatively very different. And that's then we started late in space and you're like, this is something different. We have to talk about it on a podcast.
[01:08:25] swyx: There we go. Yeah. It wasn't, it wasn't a podcast for like four months. And then, and then I had been running a discord for dev tools investors. 'cause I, I also invest in dev tools and I advise companies on deaf tools, def things. And I think it was the start of 2023 when Alessio and I were both like, you know, I think we, we need to like get more tokens out of.
[01:08:45] swyx: People, and I was running out of original sources to, to write about, so I was like, okay, I'll go get those original sources. And I think that, that's when we started the podcast. And I think it's just the chemistry between us, the, the way we spike in different ways. And also, like, honestly, the kind participation of the guests to give us their time.
[01:09:03] swyx: Like, you know, like, getting George Hoss was a big deal. And also shout out to Alessio for just cold emailing him for, for, for booking the, booking some of our biggest guests. And I'm just working really hard to try to tell the story that people can use at work. I think that there's a lot of AI podcasts out there and a lot of AI kind of forums or fireside chats with no fire.
[01:09:21] swyx: That always talk about age, like what's your AGI timeline, what's your PDoom. Very, very nice hallway conversations for freshman year but not very useful for work. And like, you know, practically like making money and like And thinking about, you know, changing the everyday lives. I think what's interesting is obviously you care about the existential safety of the human race.
[01:09:43] swyx: But in the meantime we gotta eat. So so I think that's like kind of latent space's niche. Like we explicitly don't really talk about AGI. We explicitly don't talk about Things that we're, like, a little bit too far out. Like, we don't do a ton of robotics. We don't do a ton of, like, high frequency trading.
[01:10:00] swyx: There's tons of machine learning in there, but we just don't do that. Because, like, we're like, all right, what are most software engineers gonna, gonna need? Because that's our background, and that's the audience that we serve. And I think just, like, being really clear on that audience has been, has resonated with people.
[01:10:12] swyx: Yeah, you would never expect a technical podcast to reach, like, a general audience, like, Top ten on the tech charts but I, you know, I've been surprised by that before and it's been successful. I don't know, I don't know what to say about that. I think honestly, I, I kind of have this like negative reaction towards being, being, being, being, being classified as a podcast because the podcast is downstream of ideas.
[01:10:35] swyx: And it's one mode of conversation, it's one mode of idea delivery, but you can deliver ideas on a newsletter, in person like this there's so many different ways. And so I think, I think about it more as we are trying to start or serve an industry, and that industry is the AI engineer industry, which is, which we can talk about more.
[01:10:53] swyx: Yes, let's go into that. So the AI engineer, you penned a piece called The Rise of the AI Engineer, you tweeted about it, Andrej Karpathy also responded, largely agreeing with what you said. What is an AI engineer? The AI engineer is the software engineer building with AI, enhanced by AI, And eventually it will be non human engineers writing code for you, Which I know MBU is all about.
[01:11:18] swyx: You're saying eventually the AI engineer will become a non human engineer? That will be one kind of AI engineer that people are trying to build, And is probably the most furthest away in terms of being reality. Because it's so hard. Got it. But, but there are three types of AI engineer and I just went through the three.
[01:11:33] swyx: One is AI enhanced where you like use AI products like Copilot and Cursor. And two is AI products engineer where you use the exposed AI capabilities to the end user As a software engineer, like, not doing pre training not being an ML researcher, not being an ML engineer, but just interacting with foundation models and probably APIs from foundation model labs.
[01:11:54] swyx: What's the third one? And the third one is the non human AI engineer. Got it. The fully autonomous AI engineer. Dream, you know, Coder. How long do you think it is till we get to, like, early, early versions? This is my equivalent of AGI timelines. I know, I know. You can set yourself up for this. So like, lots of active, like, I mean, I have, I have supported companies actively working on that.
[01:12:13] swyx: I think it's more useful to think about levels of autonomy. And so my answer to that is, you know, perpetually five years away until until it figures it out. No, but my actual anecdote the closest comparison we have to that is self driving. We are, we're doing this in San Francisco for those who are watching the live stream.
[01:12:32] swyx: If you haven't come to San Francisco and seen, and taken a Waymo ride just come, get a friend take a Waymo ride. I remember 2014 we covered a little bit of autos in, in my hedge fund. And I was, I remember telling a friend, I was like, self driving cars around the corner, like, this is it, like, you know, parking will be, like, parking will be a thing of the past and it didn't happen for the next 10 years.
[01:12:52] swyx: And, and, but now we, now, like, most of us in San Francisco can, can take it for granted. So I think, like, you just have to be mindful that the, the, the, the rough edges take a long time. And like, yes, it's going to work in demos, then it's going to work a little bit further out and it's just going to take a long time.
[01:13:08] swyx: The more useful mental model I have is sort of levels of autonomy. So in self driving, you have level 1, 2, 3, 4, 5 just the amount of human attention that you get. At first, like, your, your, your hands are always on 10 and 2 and you have to pay attention to the, to, to the driving every 30 seconds and eventually you can sleep in the car, right?
[01:13:25] swyx: So there's a whole spectrum of that. So what's the equivalent for that for, for coding? Keep your hands on the keyboard and then eventually you've kind of gone off. You tab to accept everything. Where are we? Oh, that's good, yeah. Yeah. Doesn't that already happen? Yeah. Approve the PR. Approve, this looks good.
[01:13:39] swyx: That's the dream that people want. It gives, it gives, really you unlock a lot of coding when people, non technical people can file issues, and then the AI engineer can sort of automatically write code, pass your tests, and if it, if it kind of works as, as, as intended. As, as advertised then you can just kind of merge it and then you, you know, 10x, 100x the number of developers in your company immediately.
[01:14:00] swyx: So that's the goal, that's the, that's the holy grail. We're not there yet but Sweep, CodeGen, there's a bunch of companies, Magic probably, are, are all working towards that. And, and so I so the TLDR, like the, the thing that we covered Alessio and I covered in the January recap that we did was that the, the basic split that people should have in their minds is the inner loop versus the outer loop for the developer.
[01:14:21] swyx: Inner loop is everything that happens in your IDE between Git commits. And outer loop is happens, is what happens when you push up your Git commit to GitHub, for example, or GitLab. And that's a nice split, which means like everything local, everything that needs to be fast is for everything that's kind of very hands on for developers.
[01:14:37] swyx: It's probably easier to automate or easier to have code assistance. That's what Copilot is, that's what, that's what all those things are. And then everything that happens autonomously when you're effectively away from the keyboard with like a GitHub issue or something that is more outer loop where you're you know, you're relying a lot more on autonomy and we are maybe, our LLMs are maybe not smart enough to do that yet.
[01:14:57] Alessio: Do you have any thoughts on
[01:14:58] swyx: kind of
[01:14:58] Alessio: the user experience and how that will change? One of the things
[01:15:01] swyx: that has happened for me, kind of looking at some of these products and playing around with things ourselves, like, You know, it sounds good to have an automated PR, then you get an automated PR and you're like, I really don't want to review like 300 lines of generated code, and like find the bug in it.
[01:15:13] swyx: Well then you have another agent that's a reviewer. That's right, but then you like tell it like, Oh, go fix it, and it comes back with 400 lines. Yes, there is a length bias to code, right? And you do have higher passing rates. In PRs. This is a documented human behavior thing, right? Send me two lines of code, I will review the shit out of that.
[01:15:33] swyx: I don't know if I can swear on this. Send me, send me 200 lines of code, looks good to me. Right? Guess what? The, the agents are going to, perfectly happy to modify, to copy that behavior from us. When we actually want them to do the opposite. So, yeah, I, I think that the GAN model of code generation is probably not going to work super well.
[01:15:50] swyx: I do think we probably need just better planning from the start. Which is, I'm just repeating the MBU thesis by the way. Just go listen to Kanjin talk about this. She's much better at it than I am. But yeah, I think I think the code review thing is going to be I think that what Codium, there are two Codiums, the Israeli one.
[01:16:10] swyx: The Israeli Codium. With the E. Yeah, Codium with the E. They still have refused to rename. I'm friends with both of them. Every month I'm like, You're like, guys, let's
[01:16:18] NLW: all come to one room. Yeah,
[01:16:19] swyx: like, you know, someone's got to fold. Codium with the E has gone, like, you've got to write the test first. Right?
[01:16:25] swyx: You write the, you write the it's like a sort of tripartite relationship. Again, this was also covered on a podcast with them, which is fantastic. Like, you interview me, you sort of through me, you interview. Like, the past avatars I've been watching the Netflix show, by the way, it's fantastic. But like, so so Codium is like, they've already thought this all the way through.
[01:16:41] swyx: They're like, okay, you write the user story, from the user story you generate all the tests, you also generate the code and you update any one of those, they all have to update together. Right? So like, once the, and, and probably the critical factor is the test generation from the story. Because everything else can just kind of bounce the heads off of those things until they pass.
[01:17:01] swyx: So you have to write good tests. It's kind of like the eat your vegetables of coding, right? Which nobody really wants to do. And so I think it's a really smart tactic to go to market by saying we automatically generate tests for you and, you know, start not great, but then get better. And eventually you get to the weakest point in the chain for the entire loop of code generation.
[01:17:25] swyx: What do you think the weakest link is? The weakest link? Yeah. It's text generation. Yeah. Yeah. Do you think there's a way to, like, are there some promising
[01:17:33] Alessio: avenues you see forward for making that actually better?
[01:17:38] swyx: For making it better. You have to have, like, good isolation, and I think proper serverless cloud environments is integral to that.
[01:17:48] swyx: I, it could be like a fly. io. It could be like a Cloudflare worker. It depends how much, how many resources your test environment needs. And effectively I was talking about this, I think with maybe Rob earlier in the audience, where every agent needs a sandbox. If you're a code agent, you need a coding sandbox, but if you're whatever, like MBU used to have this, like, sort of Minefield, Minecraft's clone that was much faster.
[01:18:12] swyx: If, if you, if you have a model of the real world, you have to go, you have to go generate some plan or some code or some whatever, test it against that real world so that you can get this iterative feedback and then get the final result back that is somewhat validated against the real world. And so, like, you need a really good sandbox.
[01:18:26] swyx: I don't think people, I, I think this is, this is a, this is an infrastructure need that humans
[01:18:31] swyx + Josh Albrecht: have had for a long time. We've never solved it for ourselves. And now we have to solve it for humans. About a thousand times larger quantity of agents than, than, than actually exists. And, and so I, I, I think, like, we eventually have to involve, evolve a lot more infrastructure.
[01:18:45] swyx + Josh Albrecht: In order to serve these things. So yeah. So, for those who don't know, like I also have so, we're talking about the rise of AI engineer. I also have previous conversations about immutable infrastructure cloud environments and that kind of stuff. And this is all of a kind. Like, like, in order to solve agents and coding agents, we're going to have to solve the other stuff too along the way.
[01:19:05] swyx + Josh Albrecht: And it's really neat for me. To see all that tie together in my DevTools work that all these themes kind of reemerge just naturally, just because everything we needed for humans, we just need a hundred times more for, for for agents.
[01:19:17] Dylan Patel: Let's talk about the AI engineer. AI engineer has become a whole thing.
[01:19:21] Dylan Patel: It's become a term and also a conference. And tell us more, and a job title, tell us more about that. What's going on there?
[01:19:31] swyx + Josh Albrecht: That is like a very vague, a very, very big cloud of things. I would just say like, I think it's an emergent industry. I've seen this happen repeatedly for, so the general term is software engineer.
[01:19:44] swyx + Josh Albrecht: Programmer. In the 70s and 80s, there would not be like senior engineer. There would just be engineering. Like you, or you, I don't think they even call themselves engineer. They don't have that. What about a member of the technical staff? Oh, yeah, MTS. Very, very, very, very elite. But yeah, so like, you know, like these striations appear when the population grows and the technical depth grows over time.
[01:20:07] swyx + Josh Albrecht: Yeah. When it starts, when it ends. Not that, not that important, and then over time it's just gonna specialize. And I've seen this happen for frontend, for DevOps, for data and I can't remember what else I listed in, in that, in that piece, But those are the main three that I was around for. And I, I see this, I saw this happening for AI engineer which is effectively, now a lot of people are arguing that there is the ML researcher, the ML engineer, who sort of pairs with the researcher sometimes they also call research engineer and then on the other side of the fence is just software engineers.
[01:20:35] swyx + Josh Albrecht: And that's how it was up till about last year. And now there's this specializing and rising class of people building AI specific software that are not any of those previous titles that I just mentioned. And that's the thesis of the AI engineer, that this is an emerging category of AI. Startups of jobs I've had people from Meta, IBM, Microsoft, OpenAI tell me that they, their title is now AI engineer.
[01:20:58] swyx + Josh Albrecht: They're hiring AI engineers. So, like, I can see that this is a trend and I think that's what Andre called out in his post that, like, just mathematically, just the, just the limitations in terms of talent, research talents and GPUs, that all these will tend to concentrate in a, in a, in a, Few labs and everyone else are just going to have to rely on them or build differentiation of products in other ways And those will be AI engineers.
[01:21:21] swyx + Josh Albrecht: So mathematically there will be more AI engineers than ML engineers. It's just the truth. Right now it's the other way. Right now the number of AI engineers is maybe 10x less. So I think that the ratio will invert and you know I think the goal of the InSpace and the goal of the conference and anything else I do is to serve that
[01:21:38] Dylan Patel: growing audience.
[01:21:41] Dylan Patel: To make the distinction clear, if I'm a software engineer And I'm like, I want to become an AI engineer. What do I have to learn? Like, what additional capabilities does that type of engineer have? Funny you say that. I think you have a blog post on this very
[01:21:53] swyx + Josh Albrecht: topic. I don't actually have a specific blog post on how to, like, change classes.
[01:21:58] swyx + Josh Albrecht: I do think I always think about these in terms of yeah, Baldur's Gate and, you know D& D rule set number 5. 1 or whatever. But yeah, so I kind of intentionally left that open to leave space for others. I think when you start an industry, you need to the specifications that work the best in industries are So minimally defined so that other people can fill in the blanks.
[01:22:19] swyx + Josh Albrecht: And I want people to fill in the blanks. I want people to disagree with me and with with themselves so that we can figure this out as a, as a group. Like I don't want to overs specify everything, you know, like that that's, that's a way, that's the only way to guarantee it, that it will fail. Um, I do have a take obviously, 'cause a lot of people are, are asking me like, where to start.
[01:22:37] swyx + Josh Albrecht: And I think basically so what, what we have is latent Space University. We just finished working on day seven today. It's a seven day email course. Where basically, like, it, it is completely designed to answer the question of, like, okay, I'm a, I'm an existing software engineer, I, like, kind of, I know how to code but I don't get all this AI stuff, I've been living under a rock, or, like, it's just too overwhelming for me, you have to, like, pick for me, or curate for me as a, as a trusted friend.
[01:22:59] swyx + Josh Albrecht: And I have one hour a day for seven days. What, what, what do you do? slot in that, in that, in that bucket. So for us, it's making, making sort of LLM API, API calls. It's me, it's image generation, it's code generation, it's audio ASR, I, I think, what's, what's ASR? Audio speech recognition?
[01:23:18] swyx + Josh Albrecht: Yeah, yeah. And then I forget, I forget what the fifth and sixth one is, but the last day is agents. And, and so basically, like, I'm just like, you know, Here are seven projects that you should do to feel like you can do anything in AI. You can't really do everything in AI just from, just from that small list.
[01:23:34] swyx + Josh Albrecht: But I think it's just like, just like anything, you have to like, go through like a set list of, of things that are basic skills that I think everyone in this industry should have to be at least conversant in. If someone, if like a boss comes to you and goes like, hey, can we build this? You don't even know if the answer is no.
[01:23:52] swyx + Josh Albrecht: So I want you to move towards from like unknown unknowns to at least known unknowns. And I think that's, that's where you start being competent as an AI engineer. So, so yeah, that's LSU, Latent Space University, just to trigger the The Tigers.
[01:24:06] Dylan Patel: So do you think in the future that people, an AI engineer is going to be someone's full time job?
[01:24:10] Dylan Patel: Like people are just going to be AI engineers? Or do you think it's going to be more of a world where I'm a software engineer, and like, 20 percent of my time, I'm using open AIs, APIs, and I'm, Working on prompt engineering and stuff like that and using
[01:24:23] swyx + Josh Albrecht: CodePilot. You just reminded me of Day6's open source models and fine tuning.
[01:24:27] swyx + Josh Albrecht: Perfect. I think it will be a spectrum. That's why I don't want to be like too definitive about it. Like we have full time front end engineers and we have part time front end engineers and you dip into that community whenever you want. But wouldn't it be nice if there was a collective name for that community so you could go find it?
[01:24:40] swyx + Josh Albrecht: You can find each other. And, like, honestly, like, that's, that's really it. Like, a lot of people, a lot of companies were pinging me for, like, Hey, I want to hire this kind of person, but you can't hire that person, but I wanted someone like that. And then people on the labor side were, were pinging me going, like, Okay, I want to do more in this space, but where do I go?
[01:24:56] swyx + Josh Albrecht: And I think just having that shelling point of, of, of what an industry title and name is, and then sort of building out that. Mythology and community and conference I think is helpful, hopefully, and I don't have any prescriptions on whether or not it's a full time job. I do think, over time, it's going to become more of a full time job.
[01:25:14] swyx + Josh Albrecht: And that's great for the people who want to do that and the companies that want to employ that. But it's absolutely, like, you can take it part time, like, you know, jobs come in many formats. Yep, yep, that
[01:25:23] Dylan Patel: makes sense. Yeah. And then you have a huge world fair coming up. Yeah. Tell me about that. So,
[01:25:31] swyx + Josh Albrecht: Part of, I think, you know, What creating an industry requires is for, to let people gather in one place.
[01:25:37] swyx + Josh Albrecht: And also for me to get high quality talks out of people. You have to create an event out of it. Otherwise they don't do the work. So so last year we did the AI Engineer Summit, which went very well. And people can see that online and we're, we're, we're very happy with how that turned out.
[01:25:53] swyx + Josh Albrecht: This year we want to go four times bigger with the World's Fair and try to reflect AI engineering as it is in 2024. I always admired two conferences in, in this respect. One is NeurIPS, which I went to last year and, and documented on, on the pod, which was fantastic. And two, which is KubeCon from the other side of my life, which is the sort of cloud registration and, and DevOps world.
[01:26:18] swyx + Josh Albrecht: So NeurIPS is the one place that you go to, to, I think it's the top conference. I mean, there's, there's others that you can kind of consider. But, yeah so, so NeurIPS is, NeurIPS is where the research sciences are the stars. The researchers are the stars, PhDs are the stars, mostly it's just PhDs on the job market, to be honest.
[01:26:34] swyx + Josh Albrecht: It's really funny
[01:26:35] Dylan Patel: to go, especially these days. Yeah, it
[01:26:37] swyx + Josh Albrecht: was really funny to go to NeurIPS and go like, And the VCs trying to back them. Yeah, there are lots, lots of VCs trying to back them. Yeah, there This year. Anyway, so in Europe, research scientists are the stars. And for, I wanted for AI engineers, for engineers to be the star.
[01:26:51] swyx + Josh Albrecht: Right, to show off their tooling and their techniques and their difficulty moving all these ideas from research into production. The other one was KubeCon, where, You could honestly just go and not attend any of the talks and just walk the floor and figure out what's going on in DevOps, which is fantastic.
[01:27:10] swyx + Josh Albrecht: Because, yeah, so, so that curation and that bringing together of, of, of an industry is what I'm going for for the conference. And yeah, it's coming in June. The most important thing, to be honest, when I, like, conceived of this whole thing was to buy the domain. So we got AI. engineer. People are like, engineer is a domain?
[01:27:27] swyx + Josh Albrecht: Yeah, and funny enough, engineer was cheaper than engineering. I don't understand why, but like that's up to the domain people.
[01:27:36] Dylan Patel: Josh, any questions on agents?
[01:27:38] Alessio: Yeah,
[01:27:39] Dylan Patel: I think maybe, you know, you have a lot
[01:27:40] swyx + Josh Albrecht: of experience and exposure talking to all these companies and founders and researchers and everyone that's on your podcast.
[01:27:47] Dylan Patel: Do you have, do you feel like you have a
[01:27:50] swyx + Josh Albrecht: good kind of perspective on some of the things that, like, some of the kind of technical issues having seen? You know, like we were just talking about, like, for coding agents, like, oh, how, you know, the value of test is really important. There are other things, like, for, you know, retrieval, like now, You know, we have these models coming out with a million context, you know, or a million tokens of context length, or ten million, like, is retrieval going to
[01:28:10] Dylan Patel: matter anymore, like,
[01:28:11] swyx + Josh Albrecht: do
[01:28:11] Dylan Patel: huge contexts matter, like,
[01:28:13] swyx + Josh Albrecht: what do you think?
[01:28:14] swyx + Josh Albrecht: Specifically about the long context thing? Sure, yeah. Because you asked a more broad question. I was going to ask a few other ones after that, so go for that one first. Yeah. That's what I was going to ask first. We can ask, yeah, okay, let's talk about long context and then the other stuff. So, for those who don't know, LongContext was kind of in the air last year, but really, really, really came into focus this year.
[01:28:33] swyx + Josh Albrecht: With Gemini 1. 5 having a million token context and saying that it was in research for 10 million tokens. And that means that you can put, you, you, you, like, no longer have to really think about, What you retrieve sorry, you no longer really think about what you have to, like, put into context.
[01:28:50] swyx + Josh Albrecht: You can just kind of throw it, throw the entire knowledge base in there, or books, or film, anything like that and that's fantastic. A lot of people are thinking that it kills RAG, and I think, like, one, that's not true, because for any kind of cost reason you you know, you still pay per token, so if you there, so basically Google is, like, perfectly happy to let you pay a million tokens every single time you make an API call, but good luck, you know, having a hundred dollar API call.
[01:29:12] swyx + Josh Albrecht: And and then the other thing, it's going to be slow. No explanation needed. And then finally, my criticism of long context is that it's also not debuggable. Like, if something goes wrong with the result, you can't do, like, the ragged decomposition of where the source of error. Like, you just have to, like, go, like, it's the Waze, bro.
[01:29:29] swyx + Josh Albrecht: Like, it's somewhere in there. Sorry. I pretty strongly agree with this. Why do you think people are making such crazy long context windows? People love to kill rag, right? It's so much Kill it, though, because it's too expensive. It's so expensive like you said. Yeah, I just think I just call it a different dimension I think it's an option that's great when it's there like when I'm prototyping I do not ever want to worry about context and I'm gonna call Stuff a few times and I don't want to run to errors I don't want to have it set up a complex retrieval system just to prototype something But once I'm done prototyping then I'll worry about all the other rag stuff And yes, I'm gonna buy some system or build some system or whatever to go do that.
[01:30:02] swyx + Josh Albrecht: I so I think it's just like An improvement in like one dimension that you need And then, but the improvements in the other dimensions also matter. And it's all needed, like this space is just going to keep growing, um, in unlimited fashion. I do think that this combined with multi modality does unlock new things.
[01:30:21] swyx + Josh Albrecht: So That's what I was going to ask about next. It's like, how important is multi modal? Like, great, you know, generating videos, sure, whatever. Okay, how many of us need to generate videos that often? It'd be cool for TV shows, sure, but like, yeah. I think it's pretty important. And the one thing that, in, when we launched the Lean Space podcast, We listed a bunch of interest areas.
[01:30:37] swyx + Josh Albrecht: So one thing I love about being explicit or intentional about our, our work is that you list the things that you're interested in and you, you list the things that you're not interested in. And people are very unwilling to, to, to have an anti interest list. One of the things that we were not interested in was multimodality last year.
[01:30:55] swyx + Josh Albrecht: Because everyone was, I was just like, okay, you can generate images and they're pretty, but like not a giant business. I was wrong. Midrani is a giant, giant, massive business that no one can get it, no one can understand or get into. But also I think being able to, to natively understand audio and video and code.
[01:31:12] swyx + Josh Albrecht: I consider code a special modality. All that is very, like, qualitatively different than translating it into English first and using English as, I don't know, like a bottleneck or pipe and then you know, applying it in LLMs. Like the ability of LLMs to reason across modalities gives you something more than you could, you know, Individually by, by, by using text as the universal interface.
[01:31:33] swyx + Josh Albrecht: So I think that's useful. So concretely what, what does that mean? It means that so I think the reference post for everyone that you should have in your head is Simon Willison's post on Gemini 1. 5's video capability. Where he basically shot a video of his bookshelf and just kind of scanning through it.
[01:31:50] swyx + Josh Albrecht: And he was able to give back a, a complete JSON list of the books and the authors and, and all the details that were visible there. Hallucinated some of it, which is, you know, another, another issue. But I think it's just like unlocks this use case that you just would not even try to code without the native video understanding capability.
[01:32:08] swyx + Josh Albrecht: And obviously, like. On a technical level, video is just a bunch of frames. So actually it's just image understanding, but image within the temporal dimension, which this month, I think, became much more of a important thing, like the integration of space and time in Transformers. I don't think anyone was really talking about that until this month, and now it's the only thing anyone can ever think about for Sora and for all the other stuff.
[01:32:30] swyx + Josh Albrecht: The last thing I'll say that, which is which is Against this trend of like every modality is important. They just, just do all the modalities. I kind of agree with Nat Friedman who actually kind of pointed out just before the Gemini thing blew up this, this, this, this month, which was like, why is it that OpenAI is pushing Dolly so hard?
[01:32:48] swyx + Josh Albrecht: Why is, why is being pushing Bing image creator? Like, it's not nec, it's not apparent that you have to create images to create a GI. But every lab just seems to want to do this, and I kind of agree that it's not on the critical path. Especially for image generation, maybe image understanding, video understanding.
[01:33:04] swyx + Josh Albrecht: Yeah, consumption. But generation, eh. Maybe we'll be wrong next year. It just catches you a bunch of flack with like, you know, culture war things. Alright, we're going to
[01:33:14] Dylan Patel: move into rapid fire Q& A, so we're going to ask you questions. We've cut
[01:33:26] Dylan Patel: the Q& A section for time, so if you want to hear the spicy questions, head over to the Thursday Nights in AI video for the full discussion.
[01:33:34] Dylan Patel - Semianalysis + Latent Space Live Show
[01:33:34] Dylan Patel: Next up, we have another former guest, Dylan Patel of Semianalysis, the inventor of the GPU rich poor divide, who did a special live show with us in March. But that means you can finally, like, side to side A B test your favorite Boba
[01:33:51] Alessio: shops?
[01:33:51] Alessio: We got Gong Cha, we got Boba Guys, we got the Lemon, whatever it's called. So, let us know what's your favorite. We also have Slido up to submit questions. We already had Dylan on the podcast, and like, this guy tweets and writes about all kinds of stuff. So we want to know what people want to know more
[01:34:07] Alessio: about.
[01:34:08] Alessio: Rather than just being self, self driven. But we'll do A state of the union, maybe? I don't know. Everybody wants to know about Grok. Everybody wants to know whether or not NVIDIA is going to zero after Grok. Everybody wants to know what's going on with AMD. We got some AMD folks in the crowd, too.
[01:34:23] Alessio: So feel free to interact at any time. This is We have
[01:34:27] swyx + Josh Albrecht: portable mics.
[01:34:27] Dylan Patel: Heckle, please. What do you sorry. Good comedians show their color when with the way they can handle the crowd when they're heckled.
[01:34:35] Alessio: Do not throw Boba. Do not throw Boba at this end. We cannot afford another podcasting setup. Awesome.
[01:34:41] Alessio: Well, welcome everybody to the Semi Analysis and Latest Space Crossover. Dylan texted me on Signal. He was like, dude, how do I easily set up a meetup? And here we are today. Well, as you might have seen, there's no name tags. There's a bunch of things that are missing. But we did our
[01:34:55] Dylan Patel: best. It was extremely easy, right?
[01:34:58] Groq
[01:34:58] Dylan Patel: Like, I text Alessio. He's like, yo, I got the spot. Okay, cool. Thanks Here's a link. Send it to people. Sent it. And then showed up. And like, there was zero other organization that I required. So
[01:35:10] Alessio: everybody's here. A lot of, a lot of Semi Analysis fans we get in the crowd everybody wants to know more about what's going on today, and Grok has definitely been the hottest thing.
[01:35:19] Alessio: We just recorded our monthly podcast today, and we didn't talk that much about Grok because we wanted you to talk more about it, and then we'll splice you into our, our monthly recap. So, let's start there.
[01:35:29] swyx + Josh Albrecht: Okay, so, You guys, you guys are the new Grok spreadsheet ers. Yeah, yeah, so, so, we, we we broke out some Grok numbers because everyone was wondering, there's two things going on, right?
[01:35:37] swyx + Josh Albrecht: One you know, how important, or how does it achieve the inference speed that it does? That, that has been demonstrated by GrokChat. And two, how does it achieve its price promise that is promised that, that is sort of the public pricing of 27 cents per million token. And there's been a lot of speculation or, you know, some numbers thrown out there.
[01:35:55] swyx + Josh Albrecht: I put out some tentative numbers and you put out different numbers. But I'll just kind of lay that as, as the, as the groundwork. Like, everyone's like very excited about essentially like five times faster. Token generation than any other LLM currently. And that unlocks interesting downstream possibilities if it's sustainable, if it's affordable.
[01:36:14] swyx + Josh Albrecht: And so I think your question, or reading your piece on Grok, which is on the screen right now, is it sustainable?
[01:36:21] Dylan Patel: So like many things, this is VC funded, including this Boba. No, I'm just kidding, I'm paying for the Bobo, so but, but Thank you semi analysis
[01:36:29] swyx + Josh Albrecht: subscribers
[01:36:31] Alessio: I hope he pays for it, I pay for it right now That's
[01:36:33] Dylan Patel: true, that's true Alessio has the IOU, right?
[01:36:36] Dylan Patel: And that's, that's all it is, but yeah, like many things, you know, they're, they're not making money off of their inference service, right? They're just throwing it out there for cheap and hoping to get business and maybe raise money off of that, and I think that's a that's a fine use case, but the question is, like, how much money are they losing?
[01:36:53] Dylan Patel: Right, and, and that's sort of what I went through breaking down in this this article that's on the screen. And it's, it's pretty clear they're like 7 to 10x off, like, break even on their inference API, which is like horrendous, like far worse than any other sort of inference API provider. So this is like a simple, simple cost thing that was pulled up.
[01:37:15] Dylan Patel: You can either inference at very high throughput, or you can inference at very high, very low latency.
[01:37:20] Dylan Patel: With GPUs, you can do both. With Grok, you can only do one. Of course, with Grok, you can do that one faster. Marginally faster than a inference latency optimized GPU server. But no one offers inference latency optimized GPU servers because you would just burn money, right? Makes no economic sense to do so.
[01:37:36] Dylan Patel: Until maybe someone's willing to pay for that. So, so Grok service, you know, on the surface looks awesome compared to everyone else's service, which is throughput optimized. And, and then when you compare to the throughput optimized scenario, right, GPUs look quite slow, but the reality is they're serving, you know, 64, 128 users at once.
[01:37:54] Dylan Patel: Right, they're, they have a batch size, right? How many users are being served at once, whereas Grok Taking 576 chips, and they're not really doing that efficiently, right? You know, they're, they're serving a far, far fewer number of users, but extremely fast. Now, that could be worthwhile if they can get their, you know, the number of users they're serving at once up, but that's extremely hard because they don't have memory on their chip, so they can't store KV cache KV cache for, you know, all the various different users.
[01:38:21] Dylan Patel: And so, so the crux of the issue is just like, hey, So, can they, can they get that performance up as much as they claim they will, right? Which is, you know, they need to get it up more than 10x, right? To, to, to make this like a reasonable benefit, right? In the meantime, NVIDIA's launching a new GPU in two weeks that'll be fun at GTC and they're constantly pushing software as well, so we'll see if, if Grok can catch up to that.
[01:38:43] Dylan Patel: But the, the current verdict is, you know, they're, they're quite far behind, but it's hopeful, you know, that, that maybe they can get there by, you know, scaling their system larger. Yeah.
[01:38:52] swyx + Josh Albrecht: I was listening back to our original episode, and you were talking about how NVIDIA basically adopted this different strategy of just leaning on networking GPUs together.
[01:39:00] swyx + Josh Albrecht: And it seems like Grok has some, like, minor version of that going on here with the Grok rack. Is it enough? Like, what's Grok's next step here, like,
[01:39:12] Dylan Patel: strategically? Yeah, that's the next step is, of course, you know, so, you know, So right now they connect 10 racks of chips together, right, and that's the system that's running on their API today, right.
[01:39:23] Dylan Patel: Whereas most people who are running, you know, Mistral are running it on two GPUs, right. So one fourth of a server. Yeah. And that rack is not you know, obviously 10 racks is pretty crazy, but they think that they can scale performance if they have this individual system be 20 racks, right? They think they can continue to scale performance extra linearly.
[01:39:42] Dylan Patel: So that'd be amazing if they could but I, I, I'm, I'm doubtful that that's gonna be something that's scalable especially for, for, you know, larger models. So there's the
[01:39:56] Alessio: chip itself, but there's also a lot of work they're doing at the compiler level. Do you have any good sense of, like, how easy it is to actually work with LPU?
[01:40:04] Alessio: Like, is that something that is going to be a bottleneck for them?
[01:40:07] Dylan Patel: So, so Ali's in the front right there, and he, he knows a ton about about VLIW architectures. But to summarize sort of his opinion, and I think many folks's, it's, it's extremely hard to To program these sorts of architectures, right?
[01:40:19] Dylan Patel: Which is why they have their compiler and so on and so forth. But, you know, it's, it's an incredible amount of work for them to stand up individual models and to get the performance up on them which is what they've been working on, right? Whereas, whereas, you know, GPUs are far more flexible, of course.
[01:40:33] Dylan Patel: And so the question is, you know, can they, can they can, can this compiler continue to extract performance? Well, theoretically, like there, there's a lot more performance to run on the hardware. But they don't have, you know, many, many things that people generally associate with, with programmable hardware.
[01:40:49] Dylan Patel: Right? They don't have buffers and, and many other things. So, so it makes it very tough to to do that. But that's what their, you know, their relatively large compiler team is working on. Yeah,
[01:40:58] swyx + Josh Albrecht: So I'm, I'm not a GPU compiler guy. But I do want to clarify my understanding from what I read. Which is a lot of catching up to do.
[01:41:05] swyx + Josh Albrecht: It is, The crux of it is some kind of speculative, like I, in the, the word that comes to mind is speculative routing of weights and, you know, and, and work that, that needs to be done, or scheduling of work across the, you know, the 10 racks of, of GPUs. Is that the, is that like the, the, the bulk of the benefit that you get from
[01:41:25] Dylan Patel: the compilation?
[01:41:26] Dylan Patel: So, so with the Grok chips, what's really interesting is like with GPUs you can do, you can issue certain instructions. And you will get a different result. Like, depending on the time, I know a lot of people in ML have, have had that experience, right? Where like, the GPU literally doesn't return the numbers it should be.
[01:41:45] Dylan Patel: And that's basically called non determinism, right? And, and, and the, and, and, with, with Grok, their chip is completely deterministic. The moment you compile it, you know exactly how long it will take to operate, right? There is no, there is no, like, deviation at all. And so, you know, they've, they're planning everything ahead of time, right, like, every instruction, like, it will complete in the time that they've planned it for.
[01:42:08] Dylan Patel: And there is no I don't know, I don't know what the best way to state this is. There's no variance there which is interesting from, like, when you look historically, they tried to push this into automotive, right? Because automotive, you know, you probably want your car to do exactly what you issued it to do.
[01:42:22] Dylan Patel: And not have, sort of, unpredictability. But yeah, I don't, sorry, I lost track of the question.
[01:42:28] swyx + Josh Albrecht: It's okay, I just wanted to understand a little bit more about, like, what people should under, should know about the compiler magic that goes on with Brock. Like, you know, like, I think, I think, from a software, like, under, like, hardware point of view that in, that intersection of, you know,
[01:42:44] Dylan Patel: So, so, so chips have like, like and I'm going to steal this from someone here in the crowd, but chips have like five, you know, sort of, there's like, when you're designing a chip, there's, there's, it's called PPA, right?
[01:42:54] Dylan Patel: Power, performance, and area, right? So it's kind of a triangle that you optimize around. And the one thing people don't realize is there's a, there's a third P that's like PPAP. And the last P is pain in the ass to program. And, and that's that is very important for like. People making AI hardware, right?
[01:43:11] Dylan Patel: Like, TPU, without the hundreds of people that work on the compiler, and JAX, and XLA, and all these sorts of things, would be a pain in the ass to program. But Google's got that, like, plumbing. Now, if you look across the ecosystem, everything else is a pain in the ass to program compared to NVIDIA, right? And, and, and this applies to the, to the Grok chip as well, right?
[01:43:31] Dylan Patel: So, yeah, question is, like, can the compiler team get performance up anywhere close to theoretical? And then, and then can they make it not a pain in the ass to support new models? Cool. We
[01:43:41] Alessio: got a question, we got a question from Ali. What's the average VLIW bundle occupancy of Grok? Bro,
[01:43:49] Dylan Patel: get out of here.
[01:43:52] Alessio: I don't know if he's setting you up, or if he
[01:43:54] Dylan Patel: wants to chime in. I think he's setting me up, I think he's setting me up. So, okay,
[01:43:58] swyx + Josh Albrecht: what is VLIW for
[01:44:00] Dylan Patel: the rest of us? It's, it's like very long instruction word is basically what it means. And, hm. So, so, GPUs are relatively simple, right? They're, they're tiny little cores, very simple instructions, there's a shitload of them, right?
[01:44:16] Dylan Patel: CPUs, you know, they have a, they have a known instruction set, right? x86. It's very complicated but people have worked on it for decades. VLIW processors are very unique in that sense, right? Like and your question, Ali, I cannot answer that question. I have no clue. Is it documented anywhere online?
[01:44:35] Dylan Patel: Anyway, so like the systolic array, right? Like there's, within the TPU, there's a bunch of stuff, but the actual matrix multiply unit, it's called the MXU, and it's a VLIW architecture as well. It's and I'm, I'm just trying to find a, yeah, I just want to find something that makes me not sound like an idiot.
[01:44:51] swyx + Josh Albrecht: Sometimes I also like to ballpark things in terms of like, like where a good middle median value should be and where like a good high value should be. Sorry. You, you, you
[01:45:03] Dylan Patel: can ballpark things like, you know, like, yeah, so, so, so, but basically like the, the point is like you're trading this is optimal, this is theoretically the most optimal architecture for performance power and area in a given, and you know, not, not specifically Grok, but VLIW in general is gonna get you closer to optimal there, but then you're giving off, you know, that, that last P, which is pain in the ass program, is, is I think the most simple way to get into it.
[01:45:27] Dylan Patel: There's like, computer architecture books about this, but it's, it's, it's a little little, little complicated, right? Yeah.
[01:45:35] Alessio: Somebody asked, there's a lot of questions, that's great. Can we talk about LPU, Cerebrus, Tenstorin, some of these other architectures. How should people think about Maxim, SRAM versus Mix versus
[01:45:49] Dylan Patel: Yeah, yeah.
[01:45:50] Dylan Patel: So there's a lot of ML hardware out there, new and old, right? There's old stuff that's trying to compete there's new stuff that's coming up, you know, companies like, like MadX and Lumerium Labs and so on and so forth, right? You know but, but, so, so there's like a continuum of like, everyone before, say, two years ago that was doing ML hardware bet in one direction, right?
[01:46:11] Dylan Patel: We're gonna make it as an architecture that is, that is has more on chip memory than NVIDIA, right? Like, that was the general bet everyone made. Right? And so like Grok made that bet, they made it to the extreme, right? They didn't have any off chip memory at all. Only on chip memory. You have, you have Cerebrists who did a similar thing except, they were like, Yeah, we're gonna have on chip memory, but we're gonna make a chip that's the size of a wafer.
[01:46:33] Dylan Patel: Right? Like literally this big. Whereas an NVIDIA chip is roughly this big, right? So it's like this big, it's the only chip in the world that's that big. But again, same bet. More on chip memory, less off chip, right? GraphCore and SambaNova made a similar bet. And, and every, basically everyone made that bet.
[01:46:49] Dylan Patel: Cause they thought that's where ML would go. Of course, models grew faster than anyone ever imagined. Yeah, than the memory that was possible. And so that, that very quickly became the wrong bet. And so now we're, you know, sort of seeing a new wave of startups that are going to bet on the other side, as well as many other, you know, architectural things because memory is not really the only architectural thing, of course.
[01:47:08] Dylan Patel: And so, like, where to, where to, like, place startups is, is very dependent on, like, Hey, what are you doing differently than NVIDIA? And is NVIDIA just going to implement that in their chip next year, right? Or, or some version of that. That's, like, pretty much the only things to think about when looking at, you know, hardware companies now.
[01:47:27] Dylan Patel: Cool.
[01:47:28] Alessio: And, yeah, I, I think the, the question is like, there's the size of the models that got outrun, but now you're doing all this work at the compiler level, but it's very transformer based, everything they're doing on the optimization side. How, how do you think about that risk? Like, do you think it's okay for like a hardware company to take like architectural risk in terms of like, yeah, we assume transformers in two years, they'll still be pretty good.
[01:47:51] Alessio: But when you're like depreciating some of this cost of our life. For five years as a buyer.
[01:47:56] Dylan Patel: Yeah, yeah, that's, that's the biggest challenge with like some of the specialized hardware, right? It's like, I know my GPUs will be useful in four years or five years. Maybe not, like, super useful, but they'll be useful for something.
[01:48:07] Dylan Patel: But, there's no way to know that my hardware is going to be able to operate on whatever new model architecture that comes out in the next few years, right? Like, I, I, I like to joke transformers are all you need. And like everything else is like a waste of time. But, you know, I'm sure something better will come.
[01:48:26] Dylan Patel: Right? And, and, you know, you gotta have like, hardware is expensive and you own it for many years. Right? So you can't just like buy whatever's best for today's workload one time and then assume that workload is gonna stay stagnant. Cause that's a recipe to have your like hardware useless as soon as like things evolve.
[01:48:43] Dylan Patel: Right? Like imagine if someone like had hardware for LTSMs and. 2016 or whatever, right? Like, LSTMs. Yeah, LSTM, sorry. You look like an idiot, right? Because now it's not gonna work for, you know, the next architecture, right? As soon as BERT came out, right? For example. So yeah, it's, it's very anything super, super specialized is always at risk of, of being sort of obsoleted and useless.
[01:49:06] Dylan Patel: And, and sort of that's, that's the, that's the thought that like, hey, like, like Graphcore, right? Their chips are. Pretty decent at GNNs, right? Graph Neural Networks. They're actually pretty decent at that. But no one cares, right? So, congratulations, right? Like, you won, you won like the shortest midget, right?
[01:49:24] swyx + Josh Albrecht: Mentioning transformers is all you need. Gives us a nice opportunity to bring out one of your old tweets, but also mention Gemini. My old
[01:49:30] Dylan Patel: tweets, I'm scared. Recent
[01:49:33] swyx + Josh Albrecht: tweets. There's a lot of people talking about, like I think you had a tweet commenting on Gemini 1. 5. And the million token context where basically everyone was saying, like, okay, we need Mamba, we need RLUKV, or we need some other alternative architecture to scale to long context.
[01:49:48] swyx + Josh Albrecht: And Google comes out and says, no, we just, we scaled transformers to 10 million tokens. Easy. We, and, you know, like, I, I think that, that kind of, like, reflects on your thesis there a
[01:49:59] Dylan Patel: little bit. I guess, yeah. I mean, I don't know if I, if I have a coherent thesis, but it's, it's sure fun to, it's Who, who think that like, I, I, I just have an intense hatred for RAG.
[01:50:11] Dylan Patel: Right, like retrieval augmented generation is, is, is like the most like, I just have an intense like innate hatred for it. Wait, wait, you retweeted me
[01:50:18] swyx + Josh Albrecht: defending RAG in the White House press release. Yeah, yeah, yeah. Okay.
[01:50:21] Dylan Patel: But it's just fun,
[01:50:22] swyx + Josh Albrecht: it's all fun and games. Yeah, yeah, yeah, it's all fun and games.
[01:50:24] Dylan Patel: Yeah.
[01:50:25] Dylan Patel: No, no, no, I retweeted, I retweeted you because you memed the White House. I don't know if y'all saw the meme. Can you pull it up? Sure. Like the, the White House the White House put out this thing about like, They're getting very opinionated with this White House. Memory safety. I think it was effectively like, C is bad and Rust is good.
[01:50:39] Dylan Patel: It was like pretty wild that the White House put that out. And I mean like, like whatever that is, so, so, So
[01:50:46] swyx + Josh Albrecht: like, they just got very opinionated about prescribing languages to people. And so then I was, I just like started editing them. So I have stopped comparing RAG with long context and fine
[01:50:54] Dylan Patel: tuning.
[01:50:55] Dylan Patel: Wait, You said I retweeted you defending it. I thought you were hating on it. And that's why I retweeted it.
[01:51:00] swyx + Josh Albrecht: It's somewhat of a defense. Because everyone was like long context is killing RAG. And then I had future LLM should be sub quadratic. That's another one. And I actually messed with the fine print as well..
[01:51:11] Alessio: Let's see power benefits of SRAM dominant
[01:51:13] Dylan Patel: Yeah, yeah. So, so that's a good question, right? So, like, SRAM is on chip memory. Everyone's just using HBM. If you don't have to go to off chip memory, that'd be really efficient, right?
[01:51:23] Dylan Patel: Cause, cause you're, you're not moving bits around. But there's always the issue of you don't have enough memory, right? So, so you still have to move bits around constantly. And so that's the, that's the question. So, yeah, sure. If you, if you can not move data around as you compute, it's going to be fantastically efficient.
[01:51:39] Dylan Patel: That isn't really not really just easy or simple to do.
[01:51:42] Alessio: What do you think is going to be harder in the future, like getting more energy at cheaper costs or like getting more of this hardware
[01:51:48] Dylan Patel: to run? Yeah, I wonder, so someone was talking about this earlier but it's like here in the crowd and I'm looking right at him but he's complaining that journalists keep saying that you know, that, that, like misreporting about how data centers, or what data centers are doing to the environment.
[01:52:03] Dylan Patel: Right? Which I thought was quite funny, right? Cause, cause they're inundated by journalists talking about data centers like destroying the world. Anyways you know, that's not quite the case, right? But yeah, I don't know, like, the, the, the power is certainly going to be hard to get, but, you know, I think, I think if you just look at history, right?
[01:52:22] Dylan Patel: Like humanity, especially America, right? Like, power, power production and usage kept skyrocketing. From like the 1700s to like 1970s, and then it kind of flatlined from there, so why can't we like go back to the like growth stage, I guess is like the whole like mantra of like accelerationists, I guess.
[01:52:40] Dylan Patel: This is EAC, yep. Well I don't think it's EAC, I think it's like, like Sam Altman like wholly believes this too, right? Yeah. And I don't think he's EAC. So, but yeah, like, like, I don't think like, it's like things, it's like something to think about, right? Like. The US is going back to growing in energy usage whereas for the last like 40 years kind of were flat on energy usage.
[01:53:00] Dylan Patel: And what does that mean, right? Like, yeah.
[01:53:04] Alessio: Fair enough. There was another question on Marvel but kind of the, I think
[01:53:07] Dylan Patel: that's it's, it's, it's definitely like one of these three guys who are on the buy side that are asking this question. What, what, what you want to know if Marvel's stock is gonna go up?
[01:53:18] Dylan Patel: Yeah. So Marvell,
[01:53:19] Alessio: the, they're, they're doing the custom music for, for grok. They also do the tri too. And the, the Google CPU. Yeah. Any other, any other chip that they're working on that people should, should keep in mind. It's like, yeah. Any needle moving and it's any stock moving .
[01:53:34] Dylan Patel: Yeah, exactly. Exactly. They're, they're working on some more stuff.
[01:53:38] Dylan Patel: Yeah. I, I'll, I'll, I'll refrain from,
[01:53:40] Alessio: yeah. All right. Let's see other grok stuff we want to get it, get through. I don't think so. Alright, most of the other ones. Your view on edge compute hardware. Any real use cases for it?
[01:53:54] Dylan Patel: Yeah, I mean, I, I I have like a really like anti edge view. Yeah, let's hear it.
[01:53:58] Dylan Patel: Like, like, so many people are like, oh, I'm going to run this model on my phone or on my laptop and. I love how much it's raining. So now I can be horrible and you people won't leave. Like, I want you to try and leave this building. Captive audience. Seriously, should I start singing? Like, there's nothing you
[01:54:17] Alessio: can do.
[01:54:18] Alessio: You definitely, I'll stop you from that.
[01:54:19] Dylan Patel: Sorry, so edge hardware, right? Like, you know, people are like, I'm going to run this model on my phone or my laptop. It makes no sense to me. Cause Current hardware is not really capable of it. So you're gonna buy new hardware, to run whatever on the edge or you're gonna just run very, very small models.
[01:54:36] Dylan Patel: But in either case, you're, you're gonna end up with like the performance is really low, And then whatever you spent to run it locally, Like if you spent it in the cloud, it could service 10x the users, right? So you kind of like, SOL in terms of like, Economics of, of running things on the edge. And then like latency is like, for, for LLMs, right, for LLMs, it's like not that big of a deal relative to, like internet latency is not that big of a deal relative to the use of the model, right?
[01:55:08] Dylan Patel: Like the actual model operating, whether it's on edge hardware or cloud hardware. And cloud hardware is so much faster. So like edge hardware is not really able to like, have a measurable, appreciable, like advantage. Over, over cloud, cloud hardware. This applies to diffusion models, this applies to LLMs of course small models will be able to run, but not, not all, yeah.
[01:55:33] Dylan Patel: Cool.
[01:55:35] Alessio: Let's see. I guess you, you can now see them. Yeah, what chance do startups like MetaX fetch, or 5. 6? Haven't you
[01:55:41] swyx + Josh Albrecht: already reviewed
[01:55:41] Dylan Patel: them? Why don't you, why don't you answer? Yeah, we, we
[01:55:43] swyx + Josh Albrecht: actually, like, we have, Connections with Maddox and Lemurian. Yeah, yeah, yeah. We haven't, no. But Gavin is
[01:55:52] Alessio: Yeah, yeah, they said they don't want to talk publicly.
[01:55:55] Alessio: Oh, okay, okay.
[01:55:57] swyx + Josh Albrecht: When they open up, we can Sure,
[01:56:00] Alessio: sure. But do you think, like, I think the two,
[01:56:02] Dylan Patel: three Answer the question! What do you think of them?
[01:56:06] Alessio: I think, kind of, there's a couple things. It's like How do the other companies innovate against them? I think when you do a new Silicon, you're like, Oh, we're going to be so much better at this thing or like much faster, much cheaper.
[01:56:18] Alessio: But there's all the other curves going down on the macro environment at the same time. So if it takes you like five years before you were like a lot better, five years later, once you take the chip out, you're only comparing yourself to the five year advancement that the major companies had to. So then it's like, okay, the, we're going to have like the C300, whatever, from, from NVIDIA.
[01:56:37] Alessio: By the time some of these chips come up.
[01:56:40] Dylan Patel: What's after Z? What do you think is after Z in the road map? Because it's X, Y, Z, Anyways Yeah, yeah, it's like the age old problem, right? Like you build a chip, it has some cool thing, cool feature, and then like, a year later, NVIDIA has it in hardware, right? Has implemented some flavor of that in hardware.
[01:57:01] Dylan Patel: Or two generations out, right? Like, what idea are you going to have that NVIDIA can't implement, is like, really the question. It's like, you have to be fundamentally different in some way that holds through for, you know, four or five years, right? That's kind of the big issue. But, you know, like, those people have some ideas that are interesting, and yeah, maybe it'll work out, right?
[01:57:21] Dylan Patel: But it's going to be hard to fight NVIDIA, who one, doesn't consider them competition, right? They're worried about, like, Google and Amazon's chip. Right, they're not, and I guess to some extent AMD's chip, but like they're not really worried about you know, MADX or Etched or Grok or, you know, Positron or any of these folks.
[01:57:39] Alessio: How much of an advantage do they have by working closely with like OpenAI folks and then already knowing where some of the architecture decisions are going? And since those companies are like the biggest buyers and users of the
[01:57:51] Dylan Patel: chips, Yeah, I mean, like, you see, like, the most important sort of AI companies are obviously going to tell hardware vendors what they want you know, open AI and, you know, so on and so forth, right?
[01:58:02] Dylan Patel: They're just going to obviously tell them what they want and the startups aren't actually going to get anywhere close to as much feedback on what to do on, like, you know, very minute, low level stuff, right? So that's, that's the, that is a difficulty, right? Some startups, like, like, Maddox obviously have people who built, or worked on the largest models, like at Google, but then other startups might not have that advantage and so they're always gonna have that issue of like, hey, how do I get the feedback, or what's changing, what do they see down the pipeline that's, that I really need to be aware of and ready for when I design my hardware.
[01:58:37] Dylan Patel: Alright.
[01:58:38] Alessio: Every hardware shortage has eventually turned into a glut. Well, that'd be true of NVIDIA chips, it's so when, but also why.
[01:58:45] Dylan Patel: Absolutely, and I'm so excited to buy like H100s for like 1, 000, guys. No, that's not 000, but Yeah, everyone's gonna buy chips, right? Like, it's just the way semiconductors work, because the supply chain takes forever to build out.
[01:58:58] Dylan Patel: And it's, it's like a really weird thing, right? Like, so, so if the backlog of chips is a year, people will order, you know, Two years worth of what they want for the next year. It is like a very common thing. It's not just like this AI cycle, but like, like, like microcontrollers, right? Like the automotive companies, they order two years worth of what they needed for one year, just so they could get enough, right?
[01:59:21] Dylan Patel: Like, this is just like what happens in semiconductors when, when lead times lengthen, the, the purchases and inventory is sort of like double. Sorry. So, so these. The, the NVIDIA GPU shortage obviously is going to be rectified. And when it is everyone's sort of double orders will become extremely apparent, right?
[01:59:42] Dylan Patel: And, you know, you, you see like random companies out of nowhere being like, Yeah, we've got 32, 000 H100s on order, or we've got 10, 000 or 5, 000. And trust, they're not all they're not all real orders for one, but I think, I think the like bubble will continue on for a long time, right, like it's not, it's not going to end like this year, right, like people, people need AI, right, like I think everyone in this audience would agree, right, like there's no, there's no like immediate like end to the, to the bubble, right.
[02:00:09] Dylan Patel: Party like we're in 1995, not like 2000. Makes sense.
[02:00:12] Alessio: What's next? Thoughts on VLIW
[02:00:16] Dylan Patel: architectures? Oh, Y, Y, sorry, sorry, Y. The Y question, yeah, yeah. I think it's just because the supply chain expands so much, and then at the same time there will be no, like, economic, like, immediate economic thing for everyone, right?
[02:00:28] Dylan Patel: Like, some companies will continue to buy, like like an OpenAI or Meta will continue to buy, but then, like, All these random startups will, or a lot of them will not be able to continue to buy, right? So then, so then that like kind of leads to like, they'll pause for a little bit, right? Or like, I think in 2018, right?
[02:00:45] Dylan Patel: Like memory pricing was extremely high. Then all of a sudden Google, Microsoft, and Amazon all agreed, I don't, you know, You know, they don't, they won't, they won't say it's together, but they basically all agreed it like, within the same week to stop ordering memory. And within like a month, the price of memory started tanking like insane amounts, right?
[02:01:06] Dylan Patel: And like people claim, you know, all sorts of reasons why that was timed extremely well. But it was like very clear and people in the financial markets were able to make trades and everything, right? People stopped buying and it's not like their demand just dried up. It's just like they had a little bit of a demand slowdown and then they had enough inventory that they could like weather until like prices tanked.
[02:01:26] Dylan Patel: Because it's such an inelastic good, right? Yeah.
[02:01:29] swyx + Josh Albrecht: Thank you very much. That's it.
[02:01:35] AI Charlie: That concludes our audio segment this weekend. But if you're listening all the way to the end, we have two bonus segments for you. A conversation with Malin Nefe, Senior Vice President of AI at Capital One. We'll be speaking at the AI Leadership Track of the AI Engineer World's Far. And the recent Latent Space Personal AI Meetup featuring a lot of new AI wearables. Bee, Based Hardware, DeepGram MLE AI, and LangChain LangFriend and LangMem, Presented by another former guest, Harrison Chase. Watch out and take care.
Latent Space Chats: NLW (Four Wars, GPT5), Josh Albrecht/Ali Rohde (TNAI), Dylan Patel/Semianalysis (Groq), Milind Naphade (Nvidia GTC), Personal AI (ft. Harrison Chase — LangFriend/LangMem)