We are hosting the AI World’s Fair in San Francisco on June 8th! You can RSVP here. Come meet fellow builders, see amazing AI tech showcases at different booths around the venue, all mixed with elements of traditional fairs: live music, drinks, games, and food! As always, you can find our next events and start your own here!
🗽 This edition of the newsletter is a double New York special:
First we’ll talk about our podcast with Linus of Notion AI (our first remote pod!)
then a field report from Paul Butler of Drifting in Space1, who recently held the world’s second AI UX meetup in NYC!
Podcast: Notion’s RenAIssance
In 2015, Simon Last and Ivan Zhao were down to their last dollars in funding. They laid off everyone else, threw out their 3 year old app, locked themselves in a tiny room in Kyoto, Japan, and wrote the beginnings of what became Notion.
Over 20 million users and a $10 billion valuation later, Simon and Ivan have done it again. While their 400 employees bonded at the 2022 company retreat, they locked themselves in a (less tiny) hotel room and wrote what become Notion AI, announced in November and “reverse prompt engineered” by swyx in December.
As we got to know the team this year, we were deeply impressed by the vision, care and ambition of Notion’s AI efforts:
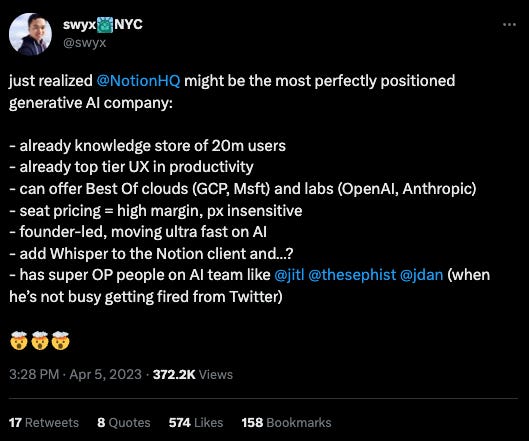
This week, they launched Notion Projects, with a powerful AI Autofill feature (first demoed at the Notion SF meetup):
Some of you may recall our very well received meetup in April, the first AI | UX meetup in the world - we first met up with Linus Lee there, and have now visited him on his home turf in the beautiful Notion NYC HQ.
Linus joined the Notion AI team in January, and is now one of the most prominent human-computer interface (HCI) voices when it comes to AI UX, with hits like:
There Are So Many PromptOps Tools And I'm Sold On None Of Them
Prompt engineering does not admit virtuosity. We need something better.
We spent a lot of time in Notion’s beautiful office in New York chatting about Notion Projects and the future of AI-assisted knowledge work. Some of the ideas that came out of it:
We see code as text files, but we understand it as a graph of references. That’s why we have features like “Go to definition”. In text we don’t have this; there’s no way to contextualize the knowledge in a doc by pulling from previous definitions. How will that evolve?
The “conceptual backwards compatibility” of everyday digital things. Buttons used to trigger mechanical systems; once we had electricity, buttons simply became binary “On/Off” switches for circuits. In the digital world, we now click a button on our mouse to click a virtual button in a UI, but there’s no mechanical or circuit work happening behind it. We still use the same abstraction because people understand button = action.
Early in a new space, a handful of companies decide on the standard interfaces. Think of the smartphone, the iPhone, and how home screens are still designed today. AI is living through this phase right now, and Notion is one of the best places in the world to do that.
Right now a lot of our AI interfaces are prompt-driven. Notion is taking full advantage of it’s incredible design to make that feel natural. If you’re using tables in Notion, you can set up an AI-autofilled Notion property so that anytime something on that database row or page changes, the AI will go back and auto-update the autofilled value.
Finally, we concluded by talking about Andy Matuschak’s conception of a structured research community, and how the emerging AI UX field needs shared tools, methods, frameworks, and core questions to professionalize or form a scenius.
Organizing the AI UX community across both US coasts has felt a little bit like this:
And we are excited to see this community blossom worldwide. (Join us!)
Show Notes
Notion
Recorded remotely at the beautiful Notion offices in NYC!
Timestamps
[00:03:30] Starting the AI / UX community
[00:10:01] Most knowledge work is not text generation
[00:16:21] Finding the right constraints and interface for AI
[00:19:06] Linus' journey to working at Notion
[00:23:29] The importance of notations and interfaces
[00:26:07] Setting interface defaults and standards
[00:32:36] The challenges of designing AI agents
[00:39:43] Notion deep dive: “Blocks”, AI, and more
[00:51:00] Prompt engineering at Notion
[01:02:00] Lightning Round
Podcast transcript comes at the end of this post.
Recap: What AI×UX Hackers are building in NYC
A guest post from Paul Butler, organizer of AI×UX NYC and BrowserTech NYC!
We recently held the first NYC AI×UX, inspired by Latent Space’s recent event in SF.
Here’s what I took away from it.
Takeaway 1: Prompt space exploration should be fun.
Three of the demos focused on tools for exploring prompt space. Each demo took a different approach, but the common thread was that exploring the prompt space of generative models can be fun:
Game designer Kyle Kukshtel demoed Dreamwalker, a DALL·E interface built in Unity, which visualizes the path of exploration through prompt space as a graph. Prompts themselves are generated by the app, with the user only guiding the direction of exploration.
Carolyn and Angelo demoed TRASHBABY, which turns prompt-space exploration into a social game. Users select a pair of images, either with their camera or from the app’s social feed, which the game uses to generate a prompt for a new image. The result is sort of like a game of telephone, where the lossiness of the photo-to-caption-to-photo pipeline becomes creative entropy in the game rather than an obstacle to overcome.
I (Paul) gave a demo exploring weighted blending of text prompts, by adjusting their relative weights with an audio mixing board. The demo serves as an example of Wirebind, a library which allows backend code to access live UI state as if it were a local variable, even in the middle of an inference loop. The tactile experience of changing prompt weights with my hands, while watching inference happen, developed my intuition for Stable Diffusion prompting in ways the usual prompt-to-result feedback loop has not.
We humans are wired to explore. Tools that have a fast feedback loop should be intrinsically fun.
Takeaway 2: Human-AI feedback loops are getting tighter.
Wand, demoed by Grant Davis, has the familiar feel of an iPad drawing app, but can turn a rough sketch into professional-looking concept art. Users can take an iterative approach, continuing to add features to an image after it has been stylized.
It’s one of the cleanest interfaces I’ve seen for tight collaboration between the user and a generative AI. I expect this sort of iterative interaction with the model is something users will come to take for granted of image generation models, the same way it has for generative text interfaces.
Takeaway 3: Data quality is no longer a blocker for private search engines.
Search engines for personal data have been slow to catch on, in part because personal data is strewn across different apps and drives, tied together by context that is hard to capture in a search index.
Brett Bejcek’s demo showed that AI can overcome these data quality issues. Rewind periodically snapshots your screen, extracts text and visual context, and provides it as context to GPT-4. The result is as if ChatGPT had been looking over your shoulder for the last year and remembered everything it saw.
I’m excited by the idea that LLMs can act as a sort of “off-road vehicle” for information retrieval, enabling useful search engines to be built for corpora that have been too messy for classical indexing and ranking algorithms.
Takeaway 4: Language learning is a killer app for conversational AI.
I love Duolingo, but I know I’m not the only one who has landed in a foreign country and quickly found that no amount of Duo streaks will translate into fluency. Chat models can pick up where vocab apps leave off, giving language learners the experience of having a conversation partner in their pocket.
Erica Du demoed Toko, which helps native Chinese speakers get better at English by talking to an AI. It’s especially useful for users who do not have regular chances to converse in English and want to get practice. Toko lets the user steer a freeform conversation, while incorporating features like grammar suggestions that a traditional teacher would give.
Takeaway 5: APIs make it easy to build high-leverage, task-specific UIs.
Two of the demos at the event were the work of developers “scratching their own itch” by building UIs on top of OpenAI APIs.
Yiren Lu of Frindle demoed a way to turn videos into markdown, in order to automate the tedious task of keeping documentation up to date. Yiren’s approach combines audio transcription and GPT to turn a video into steps, pulling frames from the video.
Ofir Press demoed a plotting UI, that allows a user to generate a chart, via matplotlib, by iteratively describing changes in natural language. Both the prompt and the code are visible on screen, so the user can alternate between writing code and prompting.
Developers have always been keen to automate repetitive workflows, but I was struck by how the simple nature of text completion APIs makes it easy to wrap an existing text model and build a UI around it for a very specific task, almost as if writing a macro. I expect we’ll see a lot more tools that enable “end-user programming” on top of APIs, allowing individual users complete the last-mile integration of LLMs into their workflow.
After the deluge of chat demos in the wake of ChatGPT, I had feared that we were hurtling towards a world in which human-computer interaction regresses back to text I/O. Above all else, the events on both coasts have convinced me that that fear is unfounded, and that UX for AI is evolving as rapidly as the foundation models themselves.
We’ll continue running events about AI, UX, and browsertech in NYC; subscribe to the browsertech digest to hear about them.
end of AI x UX NYC Recap. Thanks so much Paul!
Podcast Transcript
Alessio: Hey everyone, welcome to the Latent Space podcast. This is Alessio, partner and CTO in residence at Decibel Partners. I'm joined by my co-host Swyx, writer and editor of Latent Space. [00:00:20]
Swyx: And today we're not in our regular studio. We're actually at the Notion New York headquarters. Thanks to Linus. Welcome. [00:00:28]
Linus: Thank you. Thanks for having me. [00:00:29]
Swyx: Thanks for having us in your beautiful office. It is actually very startling how gorgeous the Notion offices are. And it's basically the same aesthetic. [00:00:38]
Linus: It's a very consistent aesthetic. It's the same aesthetic in San Francisco and the other offices. It's been for many, many years. [00:00:46]
Swyx: You take a lot of craft in everything that you guys do. Yeah. [00:00:50]
Linus: I think we can, I'm sure, talk about this more later, but there is a consistent kind of focus on taste that I think flows down from Ivan and the founders into the product. [00:00:59]
Swyx: So I'll introduce you a little bit, but also there's just, you're a very hard person to introduce because you do a lot of things. You got your BA in computer science at Berkeley. Even while you're at Berkeley, you're involved in a bunch of interesting things at Replit, CatalystX, Hack Club and Dorm Room Fund. I always love seeing people come out of Dorm Room Fund because they tend to be a very entrepreneurial. You're a product engineer at IdeaFlow, residence at Betaworks. You took a year off to do independent research and then you've finally found your home at Notion. What's one thing that people should know about you that's not on your typical LinkedIn profile? [00:01:39]
Linus: Putting me on the spot. I think, I mean, just because I have so much work kind of out there, I feel like professionally, at least, anything that you would want to know about me, you can probably dig up, but I'm a big city person, but I don't come from the city. I went to school, I grew up in Indiana, in the middle of nowhere, near Purdue University, a little suburb. I only came out to the Bay for school and then I moved to New York afterwards, which is where I'm currently. I'm in Notion, New York. But I still carry within me a kind of love and affection for small town, Indiana, small town, flyover country. [00:02:10]
Swyx: We do have a bit of indulgence in this. I'm from a small country and I think Alessio, you also kind of identified with this a little bit. Is there anything that people should know about Purdue, apart from the chickens? [00:02:24]
Linus: Purdue has one of the largest international student populations in the country, which I don't know. I don't know exactly why, but because it's a state school, the focus is a lot on STEM topics. Purdue is well known for engineering and so we tend to have a lot of folks from abroad, which is particularly rare for a university in, I don't know, that's kind of like predominantly white American and kind of Midwestern state. That makes Purdue and the surrounding sort of area kind of like a younger, more diverse international island within the, I guess, broader world that is Indiana. [00:02:58]
Swyx: Fair enough. We can always dive into sort of flyover country or, you know, small town insights later, but you and I, all three of us actually recently connected at AIUX SF, which is the first AIUX meetup, essentially which just came out of like a Twitter conversation. You and I have been involved in HCI Twitter is kind of how I think about it for a little bit and when I saw that you were in town, Geoffrey Litt was in town, Maggie Appleton in town, all on the same date, I was like, we have to have a meetup and that's how this thing was born. Well, what did it look like from your end? [00:03:30]
Linus: From my end, it looked like you did all of the work and I... [00:03:33]
Swyx: Well, you got us the Notion. Yeah, yeah. [00:03:36]
Linus: It was also in the Notion office, it was in the San Francisco one and then thereafter there was a New York one that I decided I couldn't make. But yeah, from my end it was, and I'm sure you were too, but I was really surprised by both the mixture of people that we ended up getting and the number of people that we ended up getting. There was just a lot of attention on, obviously there was a lot of attention on the technology itself of GPT and language models and so on, but I was surprised by the interest specifically on trying to come up with interfaces that were outside of the box and the people that were interested in that topic. And so we ended up having a packed house and lots of interesting demos. I've heard multiple people comment on the event afterwards that they were positively surprised by the mixture of both the ML, AI-focused people at the event as well as the interface HCI-focused people. [00:04:24]
Swyx: Yeah. I kind of see you as one of the leading, I guess, AI UX people, so I hope that we are maybe starting a new discipline, maybe. [00:04:33]
Linus: Yeah, I mean, there is this kind of growing contingency of people interested in exploring the intersection of those things, so I'm excited for where that's going to go. [00:04:41]
Swyx: I don't know if it's worth going through favorite demos. It was a little while ago, so I don't know if... [00:04:48]
Alessio: There was, I forget who made it, but there was this new document writing tool where you could apply brushes to different paragraphs. [00:04:56]
Linus: Oh, this was Amelia's. Yeah, yeah, yeah. [00:04:58]
Alessio: You could set a tone, both in terms of writer inspiration and then a tone that you wanted, and then you could drag and drop different tones into paragraphs and have the model rewrite them. It was the first time that it's not just auto-complete, there's more to it. And it's not asked in a prompt, it's this funny drag-an-emoji over it. [00:05:20]
Linus: Right. [00:05:21]
Swyx: I actually thought that you had done some kind of demo where you could select text and then augment it in different moods, but maybe it wasn't you, maybe it was just someone else [00:05:28]
Linus: I had done something similar, with slightly different building blocks. I think Amelia's demo was, there was sort of a preset palette of brushes and you apply them to text. I had built something related last year, I prototyped a way to give people sliders for different semantic attributes of text. And so you could start with a sentence, and you had a slider for length and a slider for how philosophical the text is, and a slider for how positive or negative the sentiment in the text is, and you could adjust any of them in the language model, reproduce the text. Yeah, similar, but continuous control versus distinct brushes, I think is an interesting distinction there. [00:06:03]
Swyx: I should add it for listeners, if you missed the meetup, which most people will have not seen it, we actually did a separate post with timestamps of each video, so you can look at that. [00:06:13]
Alessio: Sorry, Linus, this is unrelated, but I think you build over a hundred side projects or something like that. A hundred? [00:06:20]
Swyx: I think there's a lot of people... I know it's a hundred. [00:06:22]
Alessio: I think it's a lot of them. [00:06:23]
Swyx: A lot of them are kind of small. [00:06:25]
Alessio: Yeah, well, I mean, it still counts. I think there's a lot of people that are excited about the technology and want to hack on things. Do you have any tips on how to box, what you want to build, how do you decide what goes into it? Because all of these things, you could build so many more things on top of it. Where do you decide when you're done? [00:06:44]
Linus: So my projects actually tend to be... I think especially when people approach project building with a goal of learning, I think a common mistake is to be over-ambitious and sort of not scope things very tightly. And so a classic kind of failure mode is, you say, I'm really interested in learning how to use the GPT-4 API, and I'm also interested in vector databases, and I'm also interested in Next.js. And then you devise a project that's going to take many weeks, and you glue all these things together. And it could be a really cool idea, but then especially if you have a day job and other things that life throws you away, it's hard to actually get to a point where you can ship something. And so one of the things that I got really good at was saying, one, knowing exactly how quickly I could work, at least on the technologies that I knew well, and then only adding one new unknown thing to learn per project. So it may be that for this project, I'm going to learn how the embedding API works. Or for this project, I'm going to learn how to do vector stuff with PyTorch or something. And then I would scope things so that it fit in one chunk of time, like Friday night to Sunday night or something like that. And then I would scope the project so that I could ship something as much work as I could fit into a two-day period, so that at the end of that weekend, I could ship something. And then afterwards, if I want to add something, I have time to do it and a chance to do that. But it's already shipped, so there's already momentum, and people are using it, or I'm using it, and so there's a reason to continue building. So only adding one new unknown per project, I think, is a good trick. [00:08:14]
Swyx: I first came across you, I think, because of Monocle, which is your personal search engine. And I got very excited about it, because I always wanted a personal search engine, until I found that it was in a language that I've never seen before. [00:08:25]
Linus: Yeah, there's a towel tower of little tools and technologies that I built for myself. One of the other tricks to being really productive when you're building side projects is just to use a consistent set of tools that you know really, really well. For me, that's Go, and my language, and a couple other libraries that I've written that I know all the way down to the bottom of the stack. And then I barely have to look anything up, because I've just debugged every possible issue that could come up. And so I could get from start to finish without getting stuck in a weird bug that I've never seen before. But yeah, it's a weird stack. [00:08:58]
Swyx: It also means that you probably are not aiming for, let's say, open source glory, or whatever. Because you're not publishing in the JavaScript ecosystem. Right, right. [00:09:06]
Linus: I mean, I've written some libraries before, but a lot of my projects tend to be like, the way that I approach it is less about building something that other people are going to use en masse. And make yourself happy. Yeah, more about like, here's the thing that I built, if you want to, and often I learn something in the process of building that thing. So like with Monocle, I wrote a custom sort of full text search index. And I thought a lot of the parts of what I built was interesting. And so I just wanted other people to be able to look at it and see how it works and understand it. But the goal isn't necessarily for you to be able to replicate it and run it on your own. [00:09:36]
Swyx: Well, we can kind of dive into your other AIUX thoughts. As you've been diving in, you tend to share a lot on Twitter. And I just kind of took out some of your greatest hits. This is relevant to the demo that you picked out, Alessio. And what we're talking about, which is, most knowledge work is not a text generation task. That's funny, because a lot of what Notion AI is, is text generation right now. Maybe you want to elaborate a little bit. Yeah. [00:10:01]
Linus: I think the first time you look at something like GPT, the shape of the thing you see is like, oh, it's a thing that takes some input text and generates some output text. And so the easiest thing to build on top of that is a content generation tool. But I think there's a couple of other categories of things that you could build that are sort of progressively more useful and more interesting. And so besides content generation, which requires the minimum amount of wrapping around ChatGPT, the second tier up from that is things around knowledge, I think. So if you have, I mean, this is the hot thing with all these vector databases things going around. But if you have a lot of existing context around some knowledge about your company or about a field or all of the internet, you can use a language model as a way to search and understand things in it and combine and synthesize them. And that synthesis, I think, is useful. And at that point, I think the value that that unlocks, I think, is much greater than the value of content generation. Because most knowledge work, the artifact that you produce isn't actually about writing more words. Most knowledge work, the goal is to understand something, synthesize new things, or propose actions or other kinds of knowledge-to-knowledge tasks. And then the third category, I think, is automation. Which I think is sort of the thing that people are looking at most actively today, at least from my vantage point in the ecosystem. Things like the React prompting technique, and just in general, letting models propose actions or write code to accomplish tasks. That's also moving far beyond generating text to doing something more interesting. So much of the value of what humans sit down and do at work isn't actually in the words that they write. It's all the thinking that goes on before you write those words. So how can you get language models to contribute to those parts of work? [00:11:43]
Alessio: I think when you first tweeted about this, I don't know if you already accepted the job, but you tweeted about this, and then the next one was like, this is a NotionAI subtweet. [00:11:53]
Swyx: So I didn't realize that. [00:11:56]
Alessio: The best thing that I see is when people complain, and then they're like, okay, I'm going to go and help make the thing better. So what are some of the things that you've been thinking about? I know you talked a lot about some of the flexibility versus intuitiveness of the product. The language is really flexible, because you can say anything. And it's funny, the models never ignore you. They always respond with something. So no matter what you write, something is going to come back. Sometimes you don't know how big the space of action is, how many things you can do. So as a product builder, how do you think about the trade-offs that you're willing to take for your users? Where like, okay, I'm not going to let you be as flexible, but I'm going to create this guardrails for you. What's the process to think about the guardrails, and how you want to funnel them to the right action? [00:12:46]
Linus: Yeah, I think what this trade-off you mentioned around flexibility versus intuitiveness, I think, gets at one of the core design challenges for building products on top of language models. A lot of good interface design comes from tastefully adding the right constraints in place to guide the user towards actions that you want to take. As you add more guardrails, the obvious actions become more obvious. And one common way to make an interface more intuitive is to narrow the space of choices that the users have to make, and the number of choices that they have to make. And that intuitiveness, that source of intuitiveness from adding constraints, is kind of directly at odds with the reason that language models are so powerful and interesting, which is that they're so flexible and so general, and you can ask them to do literally anything, and they will always give you something. But most of the time, the answer isn't that high quality. And so there's kind of a distribution of, like, there are clumps of things in the action space of what a language model can do that the model's good at, and there's parts of the space where it's bad at. And so one sort of high-level framework that I have for thinking about designing with language models is, there are actions that the language model's good at, and actions that it's bad at. How do you add the right constraints carefully to guide the user and the system towards the things that the language model's good at? And then at the same time, how do you use those constraints to set the user expectations for what it's going to be good at and bad at? One way to do this is just literally to add those constraints and to set expectations. So a common example I use all the time is, if you have some AI system to answer questions from a knowledge base, there are a couple of different ways to surface that in a kind of a hypothetical product. One is, you could have a thing that looks like a chat window in a messaging app, and then you could tell the user, hey, this is for looking things up from a database. You can ask a question, then it'll look things up and give you an answer. But if something looks like a chat, and this is a lesson that's been learned over and over for anyone building chat interfaces since, like, 2014, 15, if you have anything that looks like a chat interface or a messaging app, people are going to put some, like, weird stuff in there that just don't look like the thing that you want the model to take in, because the expectation is, hey, I can use this like a messaging app, and people will send in, like, hi, hello, you know, weird questions, weird comments. Whereas if you take that same, literally the same input box, and put it in, like, a thing that looks like a search bar with, like, a search button, people are going to treat it more like a search window. And at that point, inputs look a lot more like keywords or a list of keywords or maybe questions. So the simple act of, like, contextualizing that input in different parts of an interface reset the user's expectations, which constrain the space of things that the model has to handle. And that you're kind of adding constraints, because you're really restricting your input to mostly things that look like keyword search. But because of that constraint, you can have the model fit the expectations better. You can tune the model to perform better in those settings. And it's also less confusing and perhaps more intuitive, because the user isn't stuck with this blank page syndrome problem of, okay, here's an input. What do I actually do with it? When we initially launched Notion AI, one of my common takeaways, personally, from talking to a lot of my friends who had tried it, obviously, there were a lot of people who were getting lots of value out of using it to automate writing emails or writing marketing copy. There were a ton of people who were using it to, like, write Instagram ads and then sort of paste it into the Instagram tool. But some of my friends who had tried it and did not use it as much, a frequently cited reason was, I tried it. It was cool. It was cool for the things that Notion AI was marketed for. But for my particular use case, I had a hard time figuring out exactly the way it was useful for my workflow. And I think that gets back at the problem of, it's such a general tool that just presented with a blank prompt box, it's hard to know exactly the way it could be useful to your particular use case. [00:16:21]
Alessio: What do you think is the relationship between novelty and flexibility? I feel like we're in kind of like a prompting honeymoon phase where the tools are new and then everybody just wants to do whatever they want to do. And so it's good to give these interfaces because people can explore. But if I go forward in three years, ideally, I'm not prompting anything. The UX has been built for most products to already have the intuitive, kind of like a happy path built into it. Do you think there's merit in a way? If you think about ChatGPT, if it was limited, the reason why it got so viral is people were doing things that they didn't think a computer could do, like write poems and solve riddles and all these different things. How do you think about that, especially in Notion, where Notion AI is kind of like a new product in an existing thing? How much of it for you is letting that happen and seeing how people use it? And then at some point be like, okay, we know what people want to do. The flexibility is not, it was cool before, but now we just want you to do the right things with the right UX. [00:17:27]
Linus: I think there's value in always having the most general input as an escape hatch for people who want to take advantage of that power. At this point, Notion AI has a couple of different manifestations in the product. There's the writer. There's a thing we called an AI block, which is a thing that you can always sort of re-update as a part of document. It's like a live, a little portal inside the document that an AI can write. We also have a relatively new thing called AI autofill, which lets an AI fill an entire column in a Notion database. In all of these things, speaking of adding constraints, we have a lot of suggested prompts that we've worked on and we've curated and we think work pretty well for things like summarization and writing drafts to blog posts and things. But we always leave a fully custom prompt for a few reasons. One is if you are actually a power user and you know how language models work, you can go in and write your custom prompt and if you're a power user, you want access to the power. The other is for us to be able to discover new use cases. And so one of the lovely things about working on a product like Notion is that there's such an enthusiastic and lively kind of community of ambassadors and people that are excited about trying different things and coming up with all these templates and new use cases. And having a fully custom action or prompt whenever we launch something new in AI lets those people really experiment and help us discover new ways to take advantage of AI. I think it's good in that way. There's also a sort of complement to that, which is if we wanted to use feedback data or learn from those things and help improve the way that we are prompting the model or the models that we're building, having access to that like fully diverse, fully general range of use cases helps us make sure that our models can handle the full generality of what people want to do. [00:19:06]
Swyx: I feel like we've segway’d a lot into our Notion conversation and maybe I just wanted to bridge that a little bit with your personal journey into Notion before we go into Notion proper. You spent a year kind of on a sabbatical, kind of on your own self-guided research journey and then deciding to join Notion. I think a lot of engineers out there thinking about doing this maybe don't have the internal compass that you have or don't have the guts to basically make no money for a year. Maybe just share with people how you decided to basically go on your own independent journey and what got you to join Notion in the end. [00:19:42]
Linus: Yeah, what happened? Um, yeah, so for a little bit of context for people who don't know me, I was working mostly at sort of seed stage startups as a web engineer. I actually didn't really do much AI at all for prior to my year off. And then I took all of 2022 off with less of a focus on it ended up sort of in retrospect becoming like a Linus Pivots to AI year, which was like beautifully well timed. But in the beginning of the year, there was kind of a one key motivation and then one key kind of question that I had. The motivation was that I think I was at a sort of a privileged and fortunate enough place where I felt like I had some money saved up that I had saved up explicitly to be able to take some time off and investigate my own kind of questions because I was already working on lots of side projects and I wanted to spend more time on it. I think I also at that point felt like I had enough security in the companies and folks that I knew that if I really needed a job on a short notice, I could go and I could find some work to do. So I wouldn't be completely on the streets. And so that security, I think, gave me the confidence to say, OK, let's try this kind of experiment.[00:20:52]
Maybe it'll only be for six months. Maybe it'll be for a year. I had enough money saved up to last like a year and change. And so I had planned for a year off and I had one sort of big question that I wanted to explore. Having that single question, I think, actually was really helpful for focusing the effort instead of just being like, I'm going to side project for a year, which I think would have been less productive. And that big question was, how do we evolve text interfaces forward? So, so much of knowledge work is consuming walls of text and then producing more walls of text. And text is so ubiquitous, not just in software, but just in general in the world. They're like signages and menus and books. And it's ubiquitous, but it's not very ergonomic. There's a lot of things about text interfaces that could be better. And so I wanted to explore how we could make that better. A key part of that ended up being, as I discovered, taking advantage of this new technologies that let computers make sense of text information. And so that's how I ended up sort of sliding into AI. But the motivation in the beginning was less focused on learning a new technology and more just on exploring this general question space. [00:21:53]
Swyx: Yeah. You have the quote, text is the lowest denominator, not the end game. Right, right. [00:21:58]
Linus: I mean, I think if you look at any specific domain or discipline, whether it's medicine or mathematics or software engineering, in any specific discipline where there's a narrower set of abstractions for people to work with, there are custom notations. One of the first things that I wrote in this exploration year was this piece called Notational Intelligence, where I talk about this idea that so much of, as a total sidebar, there's a whole other fascinating conversation that I would love to have at some point, maybe today, maybe later, about how to evolve a budding scene of research into a fully-fledged field. So I think AI UX is kind of in this weird stage where there's a group of interesting people that are interested in exploring this space of how do you design for this newfangled technology, and how do you take that and go and build best practices and powerful methods and tools [00:22:48]
Swyx: We should talk about that at some point. [00:22:49]
Linus: OK. But in a lot of established fields, there are notations that people use that really help them work at a slightly higher level than just raw words. So notations for describing chemicals and notations for different areas of mathematics that let people work with higher-level concepts more easily. Logic, linguistics. [00:23:07]
Swyx: Yeah. [00:23:07]
Linus: And I think it's fair to say that some large part of human intelligence, especially in these more technical domains, comes from our ability to work with notations instead of work with just the raw ideas in our heads. And text is a kind of notation. It's the most general kind of notation, but it's also, because of its generality, not super high leverage if you want to go into these specific domains. And so I wanted to try to improve on that frontier. [00:23:29]
Swyx: Yeah. You said in our show notes, one of my goals over the next few years is to ensure that we end up with interface metaphors and technical conventions that set us up for the best possible timeline for creativity and inventions ahead. So part of that is constraints. But I feel like that is one part of the equation, right? What's the other part that is more engenders creativity? [00:23:47]
Linus: Tell me a little bit about that and what you're thinking there. [00:23:51]
Swyx: It's just, I feel like, you know, we talked a little bit about how you do want to constrain, for example, the user interface to guide people towards things that language models are good at. And creative solutions do arise out of constraints. But I feel like that alone is not sufficient for people to invent things. [00:24:10]
Linus: I mean, there's a lot of directions, I think, that could go from that. The origin of that thing that you're quoting is when I decided to come help work on AI at Notion, a bunch of my friends were actually quite surprised, I think, because they had expected that I would have gone and worked… [00:24:29]
Swyx: You did switch. I was eyeing that for you. [00:24:31]
Linus: I mean, I worked at a lab or at my own company or something like that. But one of the core motivations for me joining an existing company and one that has lots of users already is this exact thing where in the aftermath of a new foundational technology emerging, there's kind of a period of a few years where the winners in the market get to decide what the default interface paradigm for the technology is. So, like, mini computers, personal computers, the winners of that market got to decide Windows are and how scrolling works and what a mouse cursor is and how text is edited. Similar with mobile, the concept of a home screen and apps and things like that, the winners of the market got to decide. And that has profound, like, I think it's difficult to understate the importance of, in those few critical years, the winning companies in the market choosing the right abstractions and the right metaphors. And AI, to me, seemed like it's at that pivotal moment where it's a technology that lots of companies are adopting. There is this well-recognized need for interface best practices. And Notion seemed like a company that had this interesting balance of it could still move quickly enough and ship and prototype quickly enough to try interesting interface ideas. But it also had enough presence in the ecosystem that if we came up with the right solution or one that we felt was right, we could push it out and learn from real users and iterate and hopefully be a part of that story of setting the defaults and setting what the dominant patterns are. [00:26:07]
Swyx: Yeah, it's a special opportunity. One of my favorite stories or facts is it was like a team of 10 people that designed the original iPhone. And so all the UX that was created there is essentially what we use as smartphones today, including predictive text, because people were finding that people were kind of missing the right letters. So they just enhanced the hit area for certain letters based on what you're typing. [00:26:28]
Linus: I mean, even just the idea of like, we should use QWERTY keyboards on tiny smartphone screens. Like that's a weird idea, right? [00:26:36]
Swyx: Yeah, QWERTY is another one. So I have RSI. So this actually affects me. QWERTY was specifically chosen to maximize travel distance, right? Like it's actually not ergonomic by design because you wanted the keyboard, the key type writers to not stick. But we don't have that anymore. We're still sticking to QWERTY. I'm still sticking to QWERTY. I could switch to the other ones. I forget. QORAC or QOMAC anytime, but I don't just because of inertia. I have another thing like this. [00:27:02]
Linus: So going even farther back, people don't really think enough about where this concept of buttons come from, right? So the concept of a push button as a thing where you press it and it activates some binary switch. I mean, buttons have existed for, like mechanical buttons have existed for a long time. But really, like this modern concept of a button that activates a binary switch really gets like popularized by the popular advent of electricity. Before the electricity, if you had a button that did something, you would have to construct a mechanical system where if you press down on a thing, it affects some other lever system that affects as like the final action. And this modern idea of a button that is just a binary switch gets popularized electricity. And at that point, a button has to work in the way that it does in like an alarm clock, because when you press down on it, there's like a spring that makes sure that the button comes back up and that it completes the circuit. And so that's the way the button works. And then when we started writing graphical interfaces, we just took that idea of a thing that could be depressed to activate a switch. All the modern buttons that we have today in software interfaces are like simulating electronic push buttons where you like press down to complete a circuit, except there's actually no circuit being completed. It's just like a square on a screen. [00:28:11]
Swyx: It's all virtualized. Right. [00:28:12]
Linus: And then you control the simulation of a button by clicking a physical button on a mouse. Except if you're on a trackpad, it's not even a physical button anymore. It's like a simulated button hardware that controls a simulated button in software. And it's also just this cascade of like conceptual backwards compatibility that gets us here. I think buttons are interesting. [00:28:32]
Alessio: Where are you on the skeuomorphic design love-hate spectrum? There's people that have like high nostalgia for like the original, you know, the YouTube icon on the iPhone with like the knobs on the TV. [00:28:42]
Linus: I think a big part of that is at least the aesthetic part of it is fashion. Like fashion taken very literally, like in the same way that like the like early like Y2K 90s aesthetic comes and goes. I think skeuomorphism as expressed in like the early iPhone or like Windows XP comes and goes. There's another aspect of this, which is the part of skeuomorphism that helps people understand and intuit software, which has less to do with skeuomorphism making things easier to understand per se and more about like, like a slightly more general version of skeuomorphism is like, there should be a consistent mental model behind an interface that is easy to grok. And then once the user has the mental model, even if it's not the full model of exactly how that system works, there should be a simplified model that the user can easily understand and then sort of like adopt and use. One of my favorite examples of this is how volume controls that are designed well often work. Like on an iPhone, when you make your iPhone volume twice as loud, the sound that comes out isn't actually like at a physical level twice as loud. It's on a log scale. When you push the volume slider up on an iPhone, the speaker uses like four times more energy, but humans perceive it as twice as loud. And so the mental model that we're working with is, okay, if I make this, this volume control slider have two times more value, it's going to sound two times louder, even though actually the underlying physics is like on a log scale. But what actually happens physically is not actually what matters. What matters is how humans perceive it in the model that I have in my head. And there, I think there are a lot of other instances where the skeuomorphism isn't actually the thing. The thing is just that there should be a consistent mental model. And often the easy, consistent mental model to reach for is the models that already exist in reality, but not always. [00:30:23]
Alessio: I think the other big topic, maybe before we dive into Notion is agents. I think that's one of the toughest interfaces to crack, mostly because, you know, the text box, everybody understands that the agent is kind of like, it's like human-like feeling, you know, where it's like, okay, I'm kind of delegating something to a human, right? I think, like, Sean, you made the example of like a Calendly, like a savvy Cal, it's like an agent, because it's scheduling on your behalf for something. [00:30:51]
Linus: That's actually a really interesting example, because it's a kind of a, it's a pretty deterministic, like there's no real AI to it, but it is agent in the sense that you're like delegating it and automate something. [00:31:01]
Swyx: Yeah, it does work without me. It's great. [00:31:03]
Alessio: So that one, we figured out. Like, we know what the scheduling interface is like. [00:31:07]
Swyx: Well, that's the state of the art now. But, you know, for example, the person I'm corresponding with still has to pick a time from my calendar, which some people dislike. Sam Lesson famously says it's a sign of disrespect. I disagree with him, but, you know, it's a point of view. There could be some intermediate AI agents that would send emails back and forth like a human person to give the other person who feels slighted that sense of respect or a personalized touch that they want. So there's always ways to push it. [00:31:39]
Alessio: Yeah, I think for me, you know, other stuff that I think about, so we were doing prep for another episode and had an agent and asked it to do like a, you know, background prep on like the background of the person. And it just couldn't quite get the format that I wanted it to be, you know, but I kept to have the only way to prompt that it's like, give it text, give a text example, give a text example. What do you think, like the interface between human and agents in the future will be like, do you still think agents are like this open ended thing that are like objective driven where you say, Hey, this is what I want to achieve versus I only trust this agent to do X. And like, this is how X is done. I'm curious because that kind of seems like a lot of mental overhead, you know, to remember each agent for each task versus like if you have an executive assistant, like they'll do a random set of tasks and you can trust them because they're a human. But I feel like with agents, we're not quite there. [00:32:36]
Swyx: Agents are hard. [00:32:36]
Linus: The design space is just so vast. Since all of the like early agent stuff came out around auto GPT, I've tried to develop some kind of a thesis around it. And I think it's just difficult because there's so many variables. One framework that I usually apply to sort of like existing chat based prompting kind of things that I think also applies just as well to agents is this duality between what you might call like trust and control. So you just now you brought up this example of you had an agent try to write some write up some prep document for an episode and it couldn't quite get the format right. And one way you could describe that is you could say, Oh, the, the agent didn't exactly do what I meant and what I had in my head. So I can't trust it to do the right job. But a different way to describe it is I have a hard time controlling exactly the output of the model and I have a hard time communicating exactly what's in my head to the model. And they're kind of two sides of the same coin. I think if you, if you can somehow provide a way to with less effort, communicate and control and constrain the model output a little bit more and constrain the behavior a little bit more, I think that would alleviate the pressure for the model to be this like fully trusted thing because there's no need for trust anymore. There's just kind of guardrails that ensure that the model does the right thing. So developing ways and interfaces for these agents to be a little more constrained in its output or maybe for the human to control its output a little bit more or behavior a little bit more, I think is a productive path. Another sort of more, more recent revelation that I had while working on this and autofill thing inside notion is the importance of zones of influence for AI agents, especially in collaborative settings. So having worked on lots of interfaces for independent work on my year off, one of the surprising lessons that I learned early on when I joined notion was that if you build a collaboration permeates everything, which is great for notion because collaborating with an AI, you reuse a lot of the same metaphors for collaborating with humans. So one nice thing about this autofill thing that also kind of applies to AI blocks, which is another thing that we have, is that you don't alleviate this problem of having to ask questions like, oh, is this document written by an AI or is this written by a human? Like this need for auditability, because the part that's written by the AI is just in like the autofilled cell or in the AI block. And you can, you can tell that's written by the AI and things outside of it, you can kind of reasonably assume that it was written by you. I think anytime you have sort of an unbounded action space for, for models like agents, it's especially important to be able to answer those questions easily and to have some sense of security that in the same way that you want to know whether your like coworker or collaborator has access to a document or has modified a document, you want to know whether an AI has permissions to access something. And if it's modified something or made some edit, you want to know that it did it. And so as a compliment to constraining the model's action space proactively, I think it's also important to communicate, have the user have an easy understanding of like, what exactly did the model do here? And I think that helps build trust as well. [00:35:39]
Swyx: Yeah. I think for auto GPT and those kinds of agents in particular, anything that is destructive, you need to prompt for, I guess, or like check with, check in with the user. I know it's overloaded now. I can't say that. You have to confirm with the user. You confirm to the user. Yeah, exactly. Yeah. Yeah. [00:35:56]
Linus: That's tough too though, because you, you don't want to stop. [00:35:59]
Swyx: Yeah. [00:35:59]
Linus: One of the, one of the benefits of automating these things that you can sort of like, in theory, you can scale them out arbitrarily. I can have like a hundred different agents working for me, but if that means I'm just spending my entire day in a deluge of notifications, that's not ideal either. [00:36:12]
Swyx: Yeah. So then it could be like a reversible, destructive thing with some kind of timeouts, a time limit. So you could reverse it within some window. I don't know. Yeah. I've been thinking about this a little bit because I've been working on a small developer agent. Right. Right. [00:36:27]
Linus: Or maybe you could like batch a group of changes and can sort of like summarize them with another AI and improve them in bulk or something. [00:36:33]
Swyx: Which is surprisingly similar to the collaboration problem. Yeah. Yeah. Yeah. Exactly. Yeah. [00:36:39]
Linus: I'm telling you, the collaboration, a lot of the problems with collaborating with humans also apply to collaborating with AI. There's a potential pitfall to that as well, which is that there are a lot of things that some of the core advantages of AI end up missing out on if you just fully anthropomorphize them into like human-like collaborators. [00:36:56]
Swyx: But yeah. Do you have a strong opinion on that? Like, do you refer to it as it? Oh yeah. [00:37:00]
Linus: I'm an it person, at least for now, in 2023. Yeah. [00:37:05]
Swyx: So that leads us nicely into introducing what Notion and Notion AI is today. Do you have a pet answer as to what is Notion? I've heard it introduced as a database, a WordPress killer, a knowledge base, a collaboration tool. What is it? Yeah. [00:37:19]
Linus: I mean, the official answer is that a Notion is a connected workspace. It has a space for your company docs, meeting notes, a wiki for all of your company notes. You can also use it to orchestrate your workflows if you're managing a project, if you have an engineering team, if you have a sales team. You can put all of those in a single Notion database. And the benefit of Notion is that all of them live in a single space where you can link to your wiki pages from your, I don't know, like onboarding docs. Or you can link to a GitHub issue through a task from your documentation on your engineering system. And all of this existing in a single place in this kind of like unified, yeah, like single workspace, I think has lots of benefits. [00:37:58]
Swyx: That's the official line. [00:37:59]
Linus: There's an asterisk that I usually enjoy diving deeper into, which is that the whole reason that this connected workspace is possible is because underlying all of this is this really cool abstraction of blocks. In Notion, everything is a block. A paragraph is a block. A bullet point is a block. But also a page is a block. And the way that Notion databases work is that a database is just a collection of pages, which are really blocks. And you can like take a paragraph and drag it into a database and it'll become a page. You can take a page inside a database and pull it out and it'll just become a link to that page. And so this core abstraction of a block that can also be a page, that can also be a row in a database, like an Excel sheet, that fluidity and this like shared abstraction across all these different areas inside Notion, I think is what really makes Notion powerful. This Lego theme, this like Lego building block theme permeates a lot of different parts of Notion. Some fans of Notion might know that when you, or when you join Notion, you get a little Lego minifigure, which has Lego building blocks for workflows. And then every year you're at Notion, you get a new block that says like you've been here for a year, you've been here for two years. And then Simon, our co-founder and CTO, has a whole crate of Lego blocks on his desk that he just likes to mess with because, you know, he's been around for a long time. But this Lego building block thing, this like shared sort of all-encompassing single abstraction that you can combine to build various different kinds of workflows, I think is really what makes Notion powerful. And one of the sort of background questions that I have for Notion AI is like, what is that kind of building block for AI? [00:39:30]
Swyx: Well, we can dive into that. So what is Notion AI? Like, so I kind of view it as like a startup within the startup. Could you describe the Notion AI team? Is this like, how seriously is Notion taking the AI wave? [00:39:43]
Linus: The most seriously? The way that Notion AI came about, as I understand it, because I joined a bit later, I think it was around October last year, all of Notion team had a little offsite. And as a part of that, Ivan and Simon kind of went into a little kind of hack weekend. And the thing that they ended up hacking on inside Notion was the very, very early prototype of Notion AI. They saw this GPT-3 thing. The early, early motivation for starting Notion, building Notion in the first place for them, was sort of grounded in this utopian end-user programming vision where software is so powerful, but there are only so many people in the world that can write programs. But everyone can benefit from having a little workspace or a little program or a little workflow tool that's programmed to just fit their use case. And so how can we build a tool that lets people customize their software tools that they use every day for their use case? And I think to them, seemed like such a critical part of facilitating that, bridging the gap between people who can code and people who need software. And so they saw that, they tried to build an initial prototype that ended up becoming the first version of Notion AI. They had a prototype in, I think, late October, early November, before Chachapiti came out and sort of evolved it over the few months. But what ended up launching was sort of in line with the initial vision, I think, of what they ended up building. And then once they had it, I think they wanted to keep pushing it. And so at this point, AI is a really key part of Notion strategy. And what we see Notion becoming going forward, in the same way that blocks and databases are a core part of Notion that helps enable workflow automation and all these important parts of running a team or collaborating with people or running your life, we think that AI is going to become an equally critical part of what Notion is. And it won't be, Notion is a cool connected workspace app, and it also has AI. It'll be that what Notion is, is databases, it has pages, it has space for your docs, and it also has this sort of comprehensive suite of AI tools that permeate everything. And one of the challenges of the AI team, which is, as you said, kind of a startup within a startup right now, is to figure out exactly what that all-permeating kind of abstraction means, which is a fascinating and difficult open problem. [00:41:57]
Alessio: How do you think about what people expect of Notion versus what you want to build in Notion? A lot of this AI technology kind of changes, you know, we talked about the relationship between text and human and how human collaborates. Do you put any constraints on yourself when it's like, okay, people expect Notion to work this way with these blocks. So maybe I have this crazy idea and I cannot really pursue it because it's there. I think it's a classic innovator's dilemma kind of thing. And I think a lot of founders out there that are in a similar position where it's like, you know, series C, series D company, it's like, you're not quite yet the super established one, you're still moving forward, but you have an existing kind of following and something that Notion stands for. How do you kind of wrangle with that? [00:42:43]
Linus: Yeah, that is in some ways a challenge and that Notion already is a kind of a thing. And so we can't just scrap everything and start over. But I think it's also, there's a blessing side of it too, in that because there are so many people using Notion in so many different ways, we understand all of the things that people want to use Notion for very well. And then so we already have a really well-defined space of problems that we want to help people solve. And that helps us. We have it with the existing Notion product and we also have it by sort of rolling out these AI things early and then watching, learning from the community what people want to do [00:43:17]
Swyx: with them. [00:43:17]
Linus: And so based on those learnings, I think it actually sort of helps us constrain the space of things we think we need to build because otherwise the design space is just so large with whatever we can do with AI and knowledge work. And so watching what people have been using Notion for and what they want to use Notion for, I think helps us constrain that space a little bit and make the problem of building AI things inside Notion a little more tractable. [00:43:36]
Swyx: I think also just observing what they naturally use things for, and it sounds like you do a bunch of user interviews where you hear people running into issues and, or describe them as, the way that I describe myself actually is, I feel like the problem is with me, that I'm not creative enough to come up with use cases to use Notion AI or any other AI. [00:43:57]
Linus: Which isn't necessarily on you, right? [00:43:59]
Swyx: Exactly. [00:43:59]
Linus: Again, like it goes way back to the early, the thing we touched on early in the conversation around like, if you have too much generality, there's not enough, there are not enough guardrails to obviously point to use cases. Blank piece of paper. [00:44:10]
Swyx: I don't know what to do with this. So I think a lot of people judge Notion AI based on what they originally saw, which is write me a blog post or do a summary or do action items. Which, fun fact, for latent space, my very, very first Hacker News hit was reverse engineering Notion AI. I actually don't know if I got it exactly right. I think I got the easy ones right. And then apparently I got the action items one really wrong. So there's some art into doing that. But also you've since launched a bunch of other products and maybe you've already hinted at AI Autofill. Maybe we can just talk a little bit about what does the scope or suite of Notion AI products have been so far and what you're launching this week? Yeah. [00:44:53]
Linus: So we have, I think, three main facets of Notion AI and Notion at the moment. We have sort of the first thing that ever launched with Notion AI, which I think that helps you write. It's, going back to earlier in the conversation, it's kind of a writing, kind of a content generation tool. If you have a document and you want to generate a summary, it helps you generate a summary, pull out action items, you can draft a blog post, you can help it improve, it's helped to improve your writings, it can help fix grammar and spelling mistakes. But under the hood, it's a fairly lightweight, a thick layer of prompts. But otherwise, it's a pretty straightforward use case of language models, right? And so there's that, a tool that helps you write documents. There's a thing called an AI block, which is a slightly more constrained version of that where one common way that we use it inside Notion is we take all of our meeting notes inside Notion. And frequently when you have a meeting and you want other people to be able to go back to it and reference it, it's nice to have a summary of that meeting. So all of our meeting notes templates, at least on the AI team, have an AI block at the top that automatically summarizes the contents of that page. And so whenever we're done with a meeting, we just press a button and it'll re-summarize that, including things like what are the core action items for every person in the meeting. And so that block, as I said before, is nice because it's a constrained space for the AI to work in, and we don't have to prompt it every single time. And then the newest member of this AI collection of features is AI autofill, which brings Notion AI to databases. So if you have a whole database of user interviews and you want to pull out what are the companies, core pain points, what are their core features, maybe what are their competitor products they use, you can just make columns. And in the same way that you write Excel formulas, you can write a little AI formula, basically, where the AI will look at the contents of the page and pull out each of these key pieces of information. The slightly new thing that autofill introduces is this idea of a more automated background [00:46:43]
Swyx: AI thing. [00:46:44]
Linus: So with Writer, the AI in your document product and the AI block, you have to always ask it to update. You have to always ask it to rewrite. But if you have a column in a database, in a Notion database, or a property in a Notion database, it would be nice if you, whenever someone went back and changed the contents of the meeting node or something updated about the page, or maybe it's a list of tasks that you have to do and the status of the task changes, you might want the summary of that task or detail of the task to update. And so anytime that you can set up an autofilled Notion property so that anytime something on that database row or page changes, the AI will go back and sort of auto-update the autofilled value. And that, I think, is a really interesting part that we might continue leading into of like, even though there's AI now tied to this particular page, it's sort of doing its own thing in the background to help automate and alleviate some of that pain of automating these things. But yeah, Writer, Blocks, and Autofill are the three sort of cornerstones we have today. [00:47:42]
Alessio: You know, there used to be this glorious time where like, Roam Research was like the hottest knowledge company out there, and then Notion built Backlinks. I don't know if we are to blame for that. No, no, but how do Backlinks play into some of this? You know, I think most AI use cases today are kind of like a single page, right? Kind of like this document. I'm helping with this. Do you see some of these tools expanding to do changes across things? So we just had Itamar from Codium on the podcast, and he talked about how agents can tie in specs for features, tests for features, and the code for the feature. So like the three entities are tied together. Like, do you see some Backlinks help AI navigate through knowledge basis of companies where like, you might have the document the product uses, but you also have the document that marketing uses to then announce it? And as you make changes, the AI can work through different pieces of it? [00:48:41]
Swyx: Definitely. [00:48:41]
Linus: If I may get a little theoretical from that. One of my favorite ideas from my last year of hacking around building text augmentations with AI for documents is this realization that, you know, when you look at code in a code editor, what it is at a very lowest level is just text files. A code file is a text file, and there are maybe functions inside of it, and it's a list of functions, but it's a text file. But the way that you understand it is not as a file, like a Word document, it's a kind of a graph.[00:49:10]
Linus: Like you have a function, you have call sites to that function, there are places where you call that function, there's a place where that function is tested, many different definitions for that function. Maybe there's a type definition that's tied to that function. So it's a kind of a graph. And if you want to understand that function, there's advantages to be able to traverse that whole graph and fully contextualize where that function is used. Same with types and same with variables. And so even though its code is represented as text files, it's actually kind of a graph. And a lot of the, of what, all of the key interfaces, interface innovations behind IDEs is helping surface that graph structure in the context of a text file. So like things like go to definition or VS Code's little window view when you like look at references. And interesting idea that I explored last year was what if you bring that to text documents? So text documents are a little more unstructured, so there's a less, there's a more fuzzy kind of graph idea. But if you're reading a textbook, if there's a new term, there's actually other places where the term is mentioned. There's probably a few places where that's defined. Maybe there's some figures that reference that term. If you have an idea, there are other parts of the document where the document might disagree with that idea or cite that idea. So there's still kind of a graph structure. It's a little more fuzzy, but there's a graph structure that ties together like a body of knowledge. And it would be cool if you had some kind of a text editor or some kind of knowledge tool that let you explore that whole graph. Or maybe if an AI could explore that whole graph. And so back to your point, I think taking advantage of not just the backlinks. Backlinks is a part of it. But the fact that all of these inside Notion, all of these pages exist in a single workspace and it's a shared context. It's a connected workspace. And you can take any idea and look up anywhere to fully contextualize what a part of your engineering system design means. Or what we know about our pitching their customer at a company. Or if I wrote down a book, what are other places where that book has been mentioned? All these graph following things, I think, are really important for contextualizing knowledge. [00:51:02]
Swyx: Part of your job at Notion is prompt engineering. You are maybe one of the more advanced prompt engineers that I know out there. And you've always commented on the state of prompt ops tooling. What is your process today? What do you wish for? There's a lot here. [00:51:19]
Linus: I mean, the prompts that are inside Notion right now, they're not complex in the sense that agent prompts are complex. But they're complex in the sense that there is even a problem as simple as summarize a [00:51:31]
Swyx: page. [00:51:31]
Linus: A page could contain anything from no information, if it's a fresh document, to a fully fledged news article. Maybe it's a meeting note. Maybe it's a bug filed by somebody at a company. The range of possible documents is huge. And then you have to distill all of it down to always generate a summary. And so describing that task to AI comprehensively is pretty hard. There are a few things that I think I ended up leaning on, as a team we ended up leaning on, for the prompt engineering part of it. I think one of the early transitions that we made was that the initial prototype for Notion AI was built on instruction following, the sort of classic instruction following models, TextWG003, and so on. And then at some point, we all switched to chat-based models, like Claude and the new ChatGPT Turbo and these models. And so that was an interesting transition. It actually kind of made few-shot prompting a little bit easier, I think, in that you could give the few-shot examples as sort of previous turns in a conversation. And then you could ask the real question as the next follow-up turn. I've come to appreciate few-shot prompting a lot more because it's difficult to fully comprehensively explain a particular task in words, but it's pretty easy to demonstrate like four or five different edge cases that you want the model to handle. And a lot of times, if there's an edge case that you want a model to handle, I think few-shot prompting is just the easiest, most reliable tool to reach for. One challenge in prompt engineering that Notion has to contend with often is we want to support all the different languages that Notion supports. And so all of our prompts have to be multilingual or compatible, which is kind of tricky because our prompts are written, our instructions are written in English. And so if you just have a naive approach, then the model tends to output in English, even when the document that you want to translate or summarize is in French. And so one way you could try to attack that problem is to tell the model, answering the language of the user's query. But it's actually a lot more effective to just give it examples of not just English documents, but maybe summarizing an English document, maybe summarize a ticket filed in French, summarize an empty document where the document's supposed to be in Korean. And so a lot of our few-shot prompt-included prompts in Notion AI tend to be very multilingual, and that helps support our non-English-speaking users. The other big part of prompt engineering is evaluation. The prompts that you exfiltrated out of Notion AI many weeks ago, surprisingly pretty spot-on, at least for the prompts that we had then, especially things like summary. But they're also outdated because we've evolved them a lot more, and we have a lot more examples. And some of our prompts are just really, really long. They're like thousands of tokens long. And so every time we go back and add an example or modify the instruction, we want to make sure that we don't regress any of the previous use cases that we've supported. And so we put a lot of effort, and we're increasingly building out internal tooling infrastructure for things like what you might call unit tests and regression tests for prompts with handwritten test cases, as well as tests that are driven more by feedback from Notion users that have chosen to share their feedback with us. [00:54:31]
Swyx: You just have a hand-rolled testing framework or use Jest or whatever, and nothing custom out there. You basically said you've looked at so many prompt ops tools and you're sold on none of them. [00:54:42]
Linus: So that tweet was from a while ago. I think there are a couple of interesting tools these days. But I think at the moment, Notion uses pretty hand-rolled tools. Nothing too heavy, but it's basically a for loop over a list of test cases. We do do quite a bit of using language models to evaluate language models. So our unit test descriptions are kind of funny because the test is literally just an input document and a query, and then we expect the model to say something. And then our qualification for whether that test passes or not is just ask the language model again, whether it looks like a reasonable summary or whether it's in the right language. [00:55:19]
Swyx: Do you have the same model? Do you have entropic-criticized OpenAI or OpenAI-criticized entropic? That's a good question. Do you worry about models being biased towards its own self? [00:55:29]
Linus: Oh, no, that's not a worry that we have. I actually don't know exactly if we use different models. If you have a fixed budget for running these tests, I think it would make sense to use more expensive models for evaluation rather than generation. But yeah, I don't remember exactly what we do there. [00:55:44]
Swyx: And then one more follow-up on, you mentioned some of your prompts are thousands of tokens. That takes away from my budget as a user. Isn't that a trade-off that's a concern? So there's a limited context window, right? Some of that is taken by you as the app designer, product designer, deciding what system prompt to provide. And then the remainder is what I as a user can give you to actually summarize as my content. In theory. [00:56:10]
Linus: I think in practice there are a couple of trends that make that an issue. So for things like generating summaries, a summary is only going to be so many tokens long. If our prompts are generating you 3,000 token summaries, the prompt is not doing its job anyway. [00:56:25]
Swyx: Yeah, but the source doc is. [00:56:27]
Linus: The source doc could be longer. So if you wanted to translate a 5,000 token document, you do have to truncate it. And there is a limitation. It's not something that we are super focused on at the moment for a couple of reasons. I think there are techniques that, if we need to, help us compress those prompts. Things like parameter-efficient fine-tuning. And also the context lengths. It seems like the dominant trend is that context lengths are getting cheaper and longer constantly. Anthropic recently announced their 100,000 token context model recently. And so I think in the longer term that's going to be taken care of anyway by the models becoming more accommodating of longer contexts. And it's more of a temporary limitation. Cool. [00:57:04]
Swyx: Shall we talk about the professionalizing of a scene? [00:57:07]
Linus: Yeah, I think one of the things that is a helpful bit of context when thinking about HCI and AI in particular is, historically, HCI and AI have been sort of competing disciplines. Competing very specifically in the sense that they often fought for the same sources of funding and the same kinds of people and attention throughout the history of computer science. HCI and AI both used to come from the same or very aligned, similar, parallel motivations of, we have computers. How do we make computers work better with humans? And one way to do it was to make the machine smarter. Another way to do it was to design better interfaces. And through the AI booms and busts, when the AI boom was happening, HCI would get less funding. And when AIs had winters, HCI would get a lot more attention because it was sort of the alternative solution. And now that we have this sort of renewed attention on how to build better interfaces for AI, I think it's interesting that it's kind of a scene now. There are podcasts like this where I get to talk about interfaces and AI. But it's definitely not a fully-fledged field. My favorite definition of sort of what distinguishes the two apart comes from Andy Matuszak, where he, I'm going to butcher the quote, but he said something to the effect of, a field has at their disposal a powerful set of established tools and methods and standards and a shared set of core questions they want to answer. And so if you look at machine learning, which is obviously a really dominant established field, if you want to answer, if you want to evaluate a model, if you want to answer, if you want to solve a particular task or build a model that solves a particular task, there are powerful methods that we have, like gradient descent and specific benchmarks, for building solutions and then re-evaluating how to do the solutions. Or if you have an even more expensive problem, there are surely attempts that have been made before and then attempts that people are making now for how to attack that problem and frameworks to think about these things. In AI and UX, I think, we're very early in the evolution of that space and that community, and there's a lot of people excited, a lot of people building, but we have yet to come up with a set of best practices and tools and methods and frameworks for thinking about these things. And those will surely arise, and as they do, I think we'll see the evolution of the field. In prompt engineering and using language models in products at large, I think that community is a little farther along. It's still very fast moving because it's really young, but there are established prompting techniques like React and distillation of larger instruction following models. And these techniques, I think, are the beginnings of best practices and powerful tools at the disposal of this language model using field. [00:59:43]
Swyx: Yeah, and mostly it's just following Riley Goodside. It's how I learn about prompting techniques. Right, right. Yeah, pioneers. But yeah, I am actually interested in this. We've recently kind of rebranded the podcast or the newsletter somewhat in towards being for this term AI engineer, which I kind of view as somewhere between machine learning researcher and software engineer, some kind of in-between mix. And I think creating the media, creating meetups, creating a de facto conference for it, creating job titles, and then I think that core set of questions that everyone wants to get better at, I think that is essentially how this starts. Yeah, yeah. Pretty excited of. [01:00:25]
Linus: Creating a space for the people that are interested to come together, I think, is a really, really key important part of it. I'm always, whenever I come back to it, I'm always amazed by how if you look at the sort of golden era of theoretical physics in the early 20th century, or the golden era of early personal computing, there are maybe like two dozen people that have contributed all of the significant ideas to that field. They all kind of know each other. I always found that really fascinating. And I think the causal relationship actually goes the other way. It's not that all those people happen to know each other. It's that because there was that core set of people that always, that were very close to each other and shared ideas often, and they were co-located, that that field is able to blossom. And so I think creating that space is really critical. [01:01:08]
Swyx: Yeah, there's a very famous photo of the Solvay conference in 1927, where Albert Einstein, Niels Bohr, Marie Curie, all these top physics names. And how many Nobel laureates are in the photo, right? Yeah, and when I tweeted it out once, people were like, I didn't know these all lived together, and they all knew each other, and they must have exchanged so many ideas. [01:01:28]
Linus: I mean, similar with artists and writers that help a new kind of period blossom. [01:01:34]
Swyx: Now, is it going to be San Francisco, New York, though? [01:01:36]
Alessio: That's a spicy question. [01:01:39]
Swyx: I don't know, we'll see. Well, we're glad to at least be a part of your world, whether it is on either coast. But it's also virtual, right? Like, we have a Discord, it's happening online as well, even if you're in a small town like Indiana. [01:01:54]
Swyx: Cool, lightning round? Awesome, yeah, let's do it. [01:01:59]
Alessio: We only got three questions for you. One is acceleration, one exploration, then a final takeaway. So the first one we always like to ask is like, what is something that happened in AI that you thought would take much longer than it has? [01:02:13]
Swyx: Price is coming down. [01:02:14]
Linus: Price is coming down and or being able to get a lot more bang for your buck. So things like GPT-3.5 Turbo being, I don't know, exactly the figure, like 10 times, 20 times cheaper. [01:02:25]
Swyx: And then having GPT, then DaVinci O3. [01:02:27]
Linus: Then DaVinci O3 per token, or the super long context clod, or MPT StoryWriter, these like long context models that take, theoretically would take a lot of compute to run, but they're sort of accessible to us now. I think they're surprising because I would have thought that before these things came out, that cost per token and scaling context length, and these were like sort of core constraints that you would have to design your AI systems around. And it ends up being like, if you just wait a few months, like OpenAI will figure out how to make these models 10 times cheaper. Or Anthropic will figure out how to make the models be able to take a million tokens. And the speed at which that's happened has been surprising and a little bit frightening, because it invalidates a lot of the assumptions that I was operating with, and I have to recalibrate. [01:03:11]
Swyx: Yeah, there's this very famous law called Wurf's Law, also known as Gates's Law, that basically says software engineers will take up whatever hardware engineers give them. And I feel like there's a parallel law right now where language model improvements, AI UX people are going to take up all the improvements that language model people will give them. So, you know, they're trying to, while the language model people are improving the costs by a single order of magnitude, you, with your Notion AI autofill, are increasing by orders of magnitude the amount of consumption that's being used. [01:03:39]
Linus: Yeah, exactly. Before the show started, we were just talking about how when I was prototyping an autofill, just to make sure that things sort of like scaled up, okay, I ended up running autofill on a database with like 6,000 pages and just summaries. And usually these are fairly long pages. I ended up running through something like two or three million tokens in a matter of like 20 minutes. [01:03:58]
Swyx: Yeah. [01:03:58]
Linus: Which is not too expensive, luckily, because the models are getting cheaper. It's going to be fine. But it is like $5 or $6, which the concept of like running a test on my computer and it spending the price of like a nice coffee is kind of a weird thing still that I'm getting used to. [01:04:13]
Swyx: And Notion AI currently is $10 a month, something like that. So there's ways to make Notion lose money. [01:04:20]
Alessio: You just get negative gross margins on that test. [01:04:24]
Linus: Not sanctioned by Notion. I mean, obviously, you should use it to, you know, improve your life and support your workflows in whatever ways that's useful. [01:04:33]
Swyx: Okay, second question is about exploration. What do you think is the most interesting unsolved question in AI? [01:04:39]
Linus: Predictability, reliability. Well, in AI broadly, I think it's much harder. But with language models specifically, I think how to build dependable systems is really important. If you ask Notion AI or if you ask ChatGPT or Claude, like maybe a bullet list of X, Y, Z, sometimes it'll make those bullets with like the Unicode center dot. Sometimes it'll make them with a dash. Sometimes it'll like add a title. Sometimes it'll like bold random things. And all of the things are fine. But it's a little jarring if every time the answer is a little stochastic. I think this is much more of a concern for when you're automating tasks or having the model make decisions by itself. Predictability, dependability, so much of the software that runs the world is sort of behind-the-scenes decision-making programs that run inside enterprises and automate systems and make decisions for people. And auditability, dependability is just so critical to all of them. One avenue of work that I'm really intrigued by is in these decision-making systems, not having the model sort of internally as a black box make decisions, but having the model synthesize code that makes decisions. So you might ask the model for things like summarization, like natural language tasks, you have to ask the model. But if you wanted to, I don't know, let's say you have a document and you want to filter out all the dates. Instead of asking the model, hey, can you grab all the dates? You can ask the model to write a regular expression that captures a particular set of date formats that you really care about. And at that point, the output of the model is a program. And the nice thing about a program is you can kind of check it. There's lots of nice things. One is it's much cheaper to run afterwards. Another is you can verify it. And the program becomes a kind of a, what in design we call a boundary object, where it's a shared thing that exists both in the sphere of the human and the sphere of the computer. And you can iterate on it to fix bugs. And you can co-evolve this object that is now like a representation of this decision that you want the model to, the computer to make. But it's auditable and dependable and reliable. And so I'm pretty bullish on co-generation and other sort of like program synthesis and program verification techniques. But using the model to write the initial program and help the people maintain the software. [01:06:36]
Swyx: Yeah, I'm so excited by that. Just in terms of reliability, I'll call out our previous guest. Rojbal. Yeah, yeah. And she's working on Guardrails AI. There's also LMQL. And then Microsoft recently put out Guidance, which is their custom language thing. Have you explored any of those? [01:06:51]
Linus: I've taken a look at all of them. I've spoken to Shreya. I think this general space of like more... Speaking of adding constraints to general systems, adding constraints, adding program verification, all of these things I think are super fascinating. I also personally like it a lot. Because before I was spending a lot of my time in AI, I spent a bunch of time looking at like programming languages and compilers and interpreters. And there is just so much amazing work that has gone into how do you build automated ways to reason about a program? Like compilers and type checkers and so on. And it would be a real shame if the whole field of program synthesis and verification just became like ask GPT-4. [01:07:30]
Swyx: But actually, it's not. [01:07:30]
Linus: Like they work together. You write the program, you synthesize the program with GPT-4 from human descriptions. And then now we have this whole set of powerful techniques that we can use to more formally understand and prove things about programs. And I think the synergy of them, I'm excited to see. [01:07:44]
Swyx: Awesome. This was great, Linus. [01:07:47]
Alessio: Our last question is always, what's one message you want everyone to remember today about the space, exciting challenges? [01:07:54]
Swyx: We were at the beginning. [01:07:57]
Linus: Maybe this is really cliche. But one thing that I always used to say about when I was working on text interfaces last year [01:08:05]
Swyx: was that I would be really disappointed [01:08:07]
Linus: if in a thousand years humans are still using the same kind of like writing tools and writing systems that we are today. Like it would be pretty surprising if we're still sort of like writing documents in the same way that we are today in a thousand years. And the language and the writing system hasn't evolved at all. If humans plan to be around for many thousands of years into the future, writing has really only been around for like two, three thousand years. And it's like sort of modern form. And we should, I think, care a lot more about building flexible, powerful tools than about backwards compatibility if we plan to be around for many more times the number of years that we've been around. And so I think whether we look at something as simple as language models or as expansive as like humans interacting with text documents, I think it's worth reminding yourself often that the things that we have today are sometimes that way for a reason but often just because an artifact of like the way that we've gotten here. And text can look very different. Language models can look very different. I personally think in a couple of years we're going to do something better than transformers. So all of these things are going to change. And I think it's important to have your eyes sort of looking over the horizon at what's coming far into the future. [01:09:24]
Swyx: Nice way to end it. [01:09:25]
Alessio: Well, thank you, Linus, for coming on. This was great. Thank you. This was lovely. [01:09:29]
Linus: Thanks for having me. [01:09:31]
Another great .space domain! We approve.
Share this post